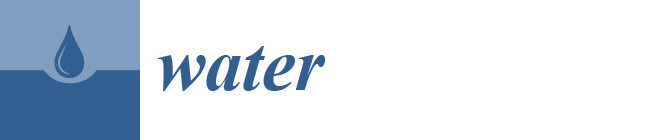
Journal Menu
► ▼ Journal Menu-
- Water Home
- Aims & Scope
- Editorial Board
- Reviewer Board
- Topical Advisory Panel
- Instructions for Authors
- Special Issues
- Topics
- Sections & Collections
- Article Processing Charge
- Indexing & Archiving
- Editor’s Choice Articles
- Most Cited & Viewed
- Journal Statistics
- Journal History
- Journal Awards
- Society Collaborations
- Conferences
- Editorial Office
Journal Browser
► ▼ Journal BrowserNeed Help?
Announcements
1 November 2024
Water | Notable Papers on Machine Learning for Water Resource Management
We invite you to read the papers below on machine learning for water resource management published in Water (ISSN: 2073-4441). The paper list is as follows.
1. “Prediction of Flow Based on a CNN-LSTM Combined Deep Learning Approach”
by Peifeng Li, Jin Zhang and Peter Krebs
Water 2022, 14(6), 993; https://doi.org/10.3390/w14060993
Available online: https://www.mdpi.com/2073-4441/14/6/993
2. “Water Level Forecasting Using Deep Learning Time-Series Analysis: A Case Study of Red River of the North”
by Vida Atashi, Hamed Taheri Gorji, Seyed Mojtaba Shahabi, Ramtin Kardan and Yeo Howe Lim
Water 2022, 14(12), 1971; https://doi.org/10.3390/w14121971
Available online: https://www.mdpi.com/2073-4441/14/12/1971
3. “Artificial Neural Networks for the Prediction of the Reference Evapotranspiration of the Peloponnese Peninsula, Greece”
by Stavroula Dimitriadou and Konstantinos G. Nikolakopoulos
Water 2022, 14(13), 2027; https://doi.org/10.3390/w14132027
Available online: https://www.mdpi.com/2073-4441/14/13/2027
4. “Comparison of Machine Learning Algorithms for Merging Gridded Satellite and Earth-Observed Precipitation Data”
by Georgia Papacharalampous, Hristos Tyralis, Anastasios Doulamis and Nikolaos Doulamis
Water 2023, 15(4), 634; https://doi.org/10.3390/w15040634
Available online: https://www.mdpi.com/2073-4441/15/4/634
5. “Improving Results of Existing Groundwater Numerical Models Using Machine Learning Techniques: A Review”
by Cristina Di Salvo
Water 2022, 14(15), 2307; https://doi.org/10.3390/w14152307
Available online: https://www.mdpi.com/2073-4441/14/15/2307
6. “Groundwater Level Prediction with Machine Learning to Support Sustainable Irrigation in Water Scarcity Regions”
by Wanru Li, Mekuanent Muluneh Finsa, Kathryn Blackmond Laskey, Paul Houser and Rupert Douglas-Bate
Water 2023, 15(19), 3473; https://doi.org/10.3390/w15193473
Available online: https://www.mdpi.com/2073-4441/15/19/3473
7. “Combining Synthetic and Observed Data to Enhance Machine Learning Model Performance for Streamflow Prediction”
by Sergio Ricardo López-Chacón, Fernando Salazar and Ernest Bladé
Water 2023, 15(11), 2020; https://doi.org/10.3390/w15112020
Available online: https://www.mdpi.com/2073-4441/15/11/2020
8. “Synchronization-Enhanced Deep Learning Early Flood Risk Predictions: The Core of Data-Driven City Digital Twins for Climate Resilience Planning”
by Maysara Ghaith, Ahmed Yosri and Wael El-Dakhakhni
Water 2022, 14(22), 3619; https://doi.org/10.3390/w14223619
Available online: https://www.mdpi.com/2073-4441/14/22/3619
9. “Using Deep Learning Algorithms for Intermittent Streamflow Prediction in the Headwaters of the Colorado River, Texas”
by Farhang Forghanparast and Ghazal Mohammadi
Water 2022, 14(19), 2972; https://doi.org/10.3390/w14192972
Available online: https://www.mdpi.com/2073-4441/14/19/2972
10. “A Machine Learning Approach for the Estimation of Total Dissolved Solids Concentration in Lake Mead Using Electrical Conductivity and Temperature”
by Godson Ebenezer Adjovu, Haroon Stephen and Sajjad Ahmad
Water 2023, 15(13), 2439; https://doi.org/10.3390/w15132439
Available online: https://www.mdpi.com/2073-4441/15/13/2439