Characterizing Regional Variability in Lung Cancer Outcomes across Ontario—A Population-Based Analysis
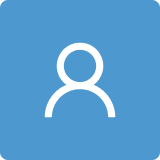
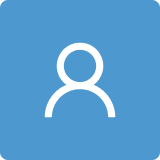
Round 1
Reviewer 1 Report
The authors have conducted a very important analysis on the topic of variations in the quality and outcomes of lung cancer care in Ontario, Canada. Although, the manuscript is very well-written, I have serious questions regarding the statistical analysis and require further information before recommending it for publication.
Major comments:
Primarily, I am concerned about their survival analysis. The authors should indicate whether they modelled (1) timeliness of treatment, (2) radiation oncology assessments, and (3) medical oncology assessments as time-varying covariates. If so, the authors should clearly state this in their methods. If not, their model is using future information which would lead to immortal time bias.
In particular, timeliness of treatment would heavily bias a Cox regression if it was not modelled as a time-varying covariate, as patients who receive delayed treatment are guaranteed to survive longer (consider that a patient who receives treatment after 180 days is guaranteed to survive for 180 days, whereas a patient who received treatment after 30 days is only guaranteed to survive for 30 days.) This may explain the inverse association between timeliness and survival, which the authors attribute to the "sicker quicker" effect.
If the authors have not used time-varying covariates, I recommend two documents that explain how to do so. The first, by Therneau et al., explains in greater detail the problem of using future information. The second, by Powell and Bagnell, explains how to implement time-varying covariates using SAS.
1. https://cran.r-project.org/web/packages/survival/vignettes/timedep.pdf
2. https://support.sas.com/resources/papers/proceedings12/168-2012.pdf
Minor comments:
- Figure 4: the authors do not provide the significance test used to compare days to treatment between LHINs.
- Page 11, first paragraph: The authors state "this graph shows the HRs for cancer specific 5-year survival calculated by Cox modeling and adjusting for..." It is not clear to me what they mean by "HRs for cancer specific 5-year survival". HRs are calculated from all available survival data. Do they mean to say that their data were censored at 5 years?
- Table 5: The authors have indicated statistically significant differences in 5-year cancer-specific survival in bold (third column) but have not indicated the test used. If significance was determined through the Cox regression, this is invalid as significant differences in hazard ratios do not always imply significant differences in survival probabilities.
Author Response
Reviewer 1:
Comment #1: The authors have conducted a very important analysis on the topic of variations in the quality and outcomes of lung cancer care in Ontario, Canada. Although, the manuscript is very well-written, I have serious questions regarding the statistical analysis and require further information before recommending it for publication.
Major comments:
Primarily, I am concerned about their survival analysis. The authors should indicate whether they modelled (1) timeliness of treatment, (2) radiation oncology assessments, and (3) medical oncology assessments as time-varying covariates. If so, the authors should clearly state this in their methods. If not, their model is using future information which would lead to immortal time bias.
In particular, timeliness of treatment would heavily bias a Cox regression if it was not modelled as a time-varying covariate, as patients who receive delayed treatment are guaranteed to survive longer (consider that a patient who receives treatment after 180 days is guaranteed to survive for 180 days, whereas a patient who received treatment after 30 days is only guaranteed to survive for 30 days.) This may explain the inverse association between timeliness and survival, which the authors attribute to the "sicker quicker" effect.
If the authors have not used time-varying covariates, I recommend two documents that explain how to do so. The first, by Therneau et al., explains in greater detail the problem of using future information. The second, by Powell and Bagnell, explains how to implement time-varying covariates using SAS.
- https://cran.r-project.org/web/packages/survival/vignettes/timedep.pdf
- https://support.sas.com/resources/papers/proceedings12/168-2012.pdf
Response #1:
Thank you for bringing this oversight to our attention. We have re-run the analysis with these three variables as time-varying covariates and updated the methods section to reflect this. Fortunately, this statistical revision did not change the direction of any associations or elements of discussion in the paper. LHIN-level variation continues to exist in the revised model. Of note, the “sicker quicker” effect appears to be stronger than previously estimated in that the HR for cancer-specific mortality in the fastest quintile of time between first abnormal imaging and initiating treatment is now >3 as opposed to 1.8 in the prior model. We agree that this important revision to the statistical methodology has significantly improved the strength of our paper.
The relevant revisions can be found in Methods Section 2.2. Statistical Approach, on Page 3, and Results Section 3.3.1, Table 4, on Pages 8-9, and Section 3.3.2, Table 5 on Page 9 and Figure 5 on Page 10.
Comment #2:
Minor comments:
Figure 4: the authors do not provide the significance test used to compare days to treatment between LHINs.
Response #2:
Thank you for bringing this to our attention. We have updated this when we re-ran the statistical analysis with time-varying covariates and indicate that we used pairwise t-test with Šidák correction for multiple comparisons and updates both the figure and figure legend.
Comment #3:
Page 11, first paragraph: The authors state "this graph shows the HRs for cancer specific 5-year survival calculated by Cox modeling and adjusting for..." It is not clear to me what they mean by "HRs for cancer specific 5-year survival". HRs are calculated from all available survival data. Do they mean to say that their data were censored at 5 years?
Response #3:
Thank you for raising this question. We can see how this wording was confusing or misleading. We did not censor the data at 5 years. We have clarified the wording of this section on Page 11 to read:
“This graph shows the HRs for cancer-specific mortality calculated by Cox modeling and adjusting for…”
Comment #4:
Table 5: The authors have indicated statistically significant differences in 5-year cancer-specific survival in bold (third column) but have not indicated the test used. If significance was determined through the Cox regression, this is invalid as significant differences in hazard ratios do not always imply significant differences in survival probabilities.
Response #4:
Thank you for bringing this to our attention. The tables have been updated to clarify that p-values are from comparison completed with Wald Chi-Square tests. This has been added to Section 2.2 of the Methods under Statistical Analysis on Page 3.
Reviewer 2 Report
I appreciate the complex interplay between patient, disease and system factors - and also understand that these factors work together to generate inequities.
It would be useful if the authors used a theoretical model or framework to situate the findings - currently everything reads disparately, and it is hard to draw conclusions or linkages. It also doesn't help that the authors use the terms disparity and inequity interchangeably, and also do not explain the social structural inequities that underpin the observations. Consider using Taplin et al (2012), Understanding and Influencing Multilevel Factors Across the Cancer Care Continuum or consider applying intersectionality to expand on the observed phenomenon.
I am also critical of the call to increase smoking cessation efforts in lower income neighborhoods and/or increase lung cancer screening efforts - there is research which specifically illustrates that this is not so simple - and that choice to undergo lung cancer screening and ability to quit smoking are strongly linked to social location. This contextualisation is missing in the analysis or calls for further action.
In summary, this is a good work - it can be enhanced by:
1. contextualising the findings using a framework
2. critically describing underpinning inequities.
Author Response
Comment #1:
I appreciate the complex interplay between patient, disease and system factors - and also understand that these factors work together to generate inequities.
It would be useful if the authors used a theoretical model or framework to situate the findings - currently everything reads disparately, and it is hard to draw conclusions or linkages. It also doesn't help that the authors use the terms disparity and inequity interchangeably, and also do not explain the social structural inequities that underpin the observations. Consider using Taplin et al (2012), Understanding and Influencing Multilevel Factors Across the Cancer Care Continuum or consider applying intersectionality to expand on the observed phenomenon.
Response #1:
We thank the reviewer for their thoughts and insights. We have added some of this essential contextualisation to the discussion of the manuscript. We agree that there are complex factors at play in the delivery of healthcare and these factors have a major impact on patient care. We feel our study further adds to this collection of literature, highlighting that differences in patient populations amongst healthcare regions did not explain the disparities in care outcomes and that likely each region has unique needs.
To address this comment, we have made the following revisions:
Page 13, Paragraph 3: “Overall, the observed variability in LC survival outcomes as well as the significant variability in patient, disease, and system factors across health regions in Ontario suggests that there is no single strategy that will improve LC outcomes across the province. Rather, the findings from this study highlight the need for regional quality improvement strategies that target the multitude of specific barriers faced by individual health regions. As underscored by Taplin et al., to achieve healthcare delivery that satisfies all domains of healthcare quality, change is required at multiple levels of the system. Ignoring the complexity of the multilevel environment in which care occurs does not achieve the desired improvements in care. Improvement efforts will need to be situated within a framework such as that suggested by Taplin et al that recognizes the need to consider care as a process in a dynamic system and the need to influence multiple levels of the system to achieve improved quality of cancer care and improved cancer-related health outcomes.”
Page 13, Paragraph 4 …”As such, quality improvement efforts will need to consider the multiple unique system barriers affecting survival in each health region. A framework such as that by Taplin et al can help in the design of effective and targeted improvement strategies that address multilevel influences on the cancer care continuum.”
Page 13, Paragraph 1: “In terms of possible additional contributing factors, there are many complexities in the medical system for which it is difficult to account for with database analysis. For example, while we did not show improved survival with more timely care, we are likely not capturing for all contacts with the healthcare system that exist along the continuum of patient care As well, patient care that included multidisciplinary discussion or care within diagnostic assessment pathway models could not be captured but has been shown to improve cancer-specific survival.[29,30] The interplay amongst these multiple layers of the healthcare system and transitions of care are an essential component to quality improvement research.[31] Studying the care systems in areas with the best survival may lead to improvement in survival across regions.”
We have also sought to improve our use of the terms disparity and inequity to address the reviewer’s comment.
Comment #2:
I am also critical of the call to increase smoking cessation efforts in lower income neighborhoods and/or increase lung cancer screening efforts - there is research which specifically illustrates that this is not so simple - and that choice to undergo lung cancer screening and ability to quit smoking are strongly linked to social location. This contextualisation is missing in the analysis or calls for further action.
Response #2:
Thank you for this comment. We agree that additional contextualization is required and that implementation of strategies such as smoking cessation efforts and lung cancer screening are complicated by the interconnectedness with other factors that influence health inequity. To address this, we have changed the wording of the Discussion on Page 12, Paragraph 2 to read as follows:
“Furthermore, there is a complex inter-relatedness between smoking and other factors that are associated with health inequity, such as immigrant status, aboriginal ancestry, and substance use, which may in turn impact cancer risk and survival outcomes. While efforts such as smoking cessation or early LC diagnosis with screening programs may be impactful in improving overall cancer outcomes, the implementation of such efforts remains a challenge due to the complex associations with other barriers to care delivery and interconnectedness with the aforementioned factors associated with health inequities.”
Additionally, we removed the part of the Discussion on Page 13 that speaks to the call to action for smoking cessation and lung cancer screening and replaced this with heightened discussion on the complex interplays between various system factors influencing care outcomes as outlined in Response #1.
Comment #3:
In summary, this is a good work - it can be enhanced by:
- contextualising the findings using a framework
- critically describing underpinning inequities.
Response #3:
We thank the reviewer for their positive comments. We have revised the manuscript to provide further contextualization of the findings with the framework proposed by Taplin et al (2012) and more critically described in complex interrelatedness of factors influencing inequity in cancer outcomes. We believe that these revisions have strengthened the message of our paper.
Round 2
Reviewer 1 Report
No further comments - well done.
Author Response
Thank you very much.
Reviewer 2 Report
Thank you for making the suggested changes.
Author Response
Thank you for your suggestions. I feel it has made our paper stronger.