Current Trends and Future Perspectives on Computer Vision and Pattern Recognition
A special issue of Applied Sciences (ISSN 2076-3417). This special issue belongs to the section "Computing and Artificial Intelligence".
Deadline for manuscript submissions: closed (31 July 2023) | Viewed by 25865
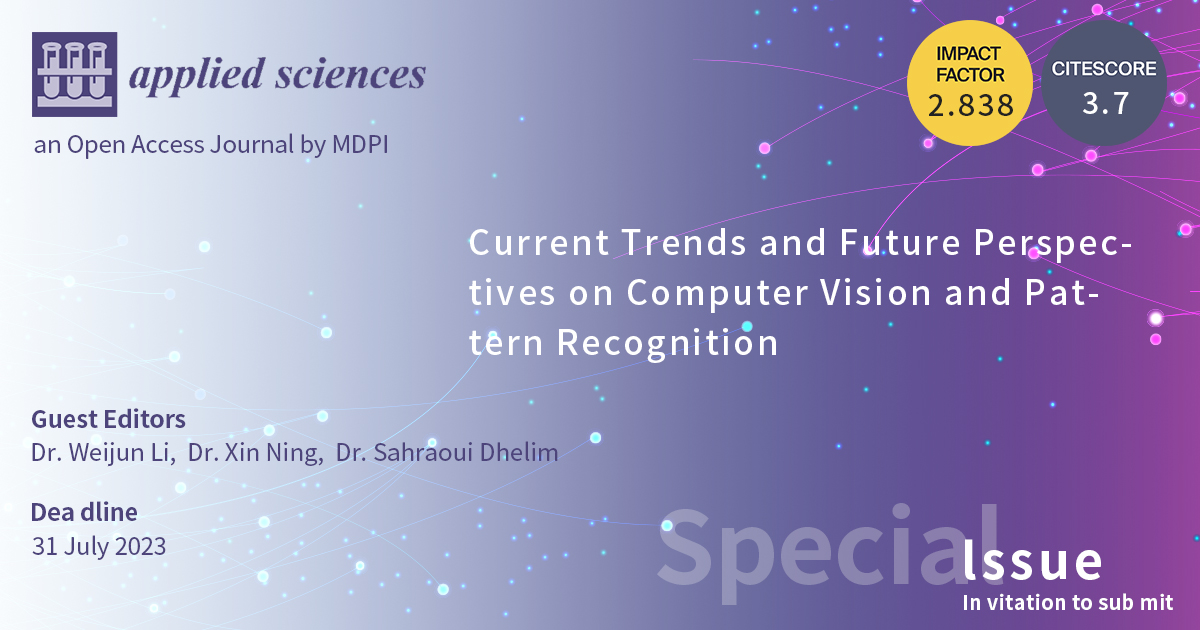
Special Issue Editors
Interests: pattern recognition; computer vision
2. Center of Materials Science and Optoelectronics Engineering School of Integrated Circuits, University of Chinese Academy of Sciences, Beijing 100049, China
Interests: pattern recognition; image classification; neural network; convolutional network; computer vision; object detection
Special Issues, Collections and Topics in MDPI journals
Interests: IoT; social computing; intelligent transportation systems; IoT; social networks analysis; mobile edge computing
Special Issues, Collections and Topics in MDPI journals
Special Issue Information
Dear Colleagues,
Recent advancements in Computer Vision and Pattern Recognition have accelerated the development of intelligent applications for numerous industries and domains. Such solutions are not only seamlessly integrated in the environment, but typically have large adaptability for unexpected conditions, which increases their usefulness for real-world problems. Recent advances in Computer Vision and Pattern Recognition have had many successes but also have several limitations and there is limited understanding of their inner workings. It is remains a major challenge in the deployment of Computer Vision and Pattern Recognition algorithms in real-world scenarios. Therefore, this paper, on the Current Trends and Future Perspectives on Computer Vision and Pattern Recognition, seeks to collect the most recent approaches and findings, as well as discuss the current challenges of Computer Vision and Pattern Recognition solutions for a wide variety of applications. We expect this Special Issue to tackle the research concerns in the closely linked fields of Computer Vision and Pattern Recognition, such as Machine Learning, Data Mining, Computer Vision and Image Processing. We encourage interdisciplinary study and application in these fields.
Important new theories, methods, applications and systems in emerging areas of Computer Vision and Pattern Recognition are welcome high-quality submissions. The topics of interest include, but are not limited to:
- Interpretable Machine Learning for Computer Vision;
- Computer vision theory;
- Semi-supervised, weakly supervised and unsupervised learning frameworks for Pattern Recognition systems;
- Embodied vision: active agents, simulation;
- Automated Deep Learning, including one or multiple stages of the machine learning process (e.g., data pre-processing, network architecture selection, hyper-parameter optimisation);
- 3D from multi-view, sensors and single images;
- Automated Deep Learning, including one or multiple stages of the machine learning process (e.g., data pre-processing, network architecture selection, hyper-parameter optimisation);
- Multimodal learning;
- Ethics/Privacy issues in deploying Pattern Recognition-based systems;
- Virtual and augmented reality content and systems;
- Benchmarks of current Pattern-Recognition-based solutions for real-world problems;
Dr. Weijun Li
Dr. Xin Ning
Dr. Sahraoui Dhelim
Guest Editors
Manuscript Submission Information
Manuscripts should be submitted online at www.mdpi.com by registering and logging in to this website. Once you are registered, click here to go to the submission form. Manuscripts can be submitted until the deadline. All submissions that pass pre-check are peer-reviewed. Accepted papers will be published continuously in the journal (as soon as accepted) and will be listed together on the special issue website. Research articles, review articles as well as short communications are invited. For planned papers, a title and short abstract (about 100 words) can be sent to the Editorial Office for announcement on this website.
Submitted manuscripts should not have been published previously, nor be under consideration for publication elsewhere (except conference proceedings papers). All manuscripts are thoroughly refereed through a single-blind peer-review process. A guide for authors and other relevant information for submission of manuscripts is available on the Instructions for Authors page. Applied Sciences is an international peer-reviewed open access semimonthly journal published by MDPI.
Please visit the Instructions for Authors page before submitting a manuscript. The Article Processing Charge (APC) for publication in this open access journal is 2400 CHF (Swiss Francs). Submitted papers should be well formatted and use good English. Authors may use MDPI's English editing service prior to publication or during author revisions.
Keywords
- pattern-recognition systems
- machine learning
- computer vision
- virtual reality
- object detection and classfication
Benefits of Publishing in a Special Issue
- Ease of navigation: Grouping papers by topic helps scholars navigate broad scope journals more efficiently.
- Greater discoverability: Special Issues support the reach and impact of scientific research. Articles in Special Issues are more discoverable and cited more frequently.
- Expansion of research network: Special Issues facilitate connections among authors, fostering scientific collaborations.
- External promotion: Articles in Special Issues are often promoted through the journal's social media, increasing their visibility.
- e-Book format: Special Issues with more than 10 articles can be published as dedicated e-books, ensuring wide and rapid dissemination.
Further information on MDPI's Special Issue polices can be found here.