Multi-Resolution Discrete Cosine Transform Fusion Technique Face Recognition Model
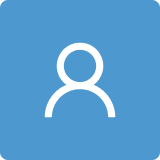
Round 1
Reviewer 1 Report
The manuscript proposes a multi-resolution discrete cosine transform fusion technology, the unique fusion feature level face recognition model (fflfrm), including face detection, feature extraction, feature fusion and face classification.
According to the image features (low resolution problem and occlusion problem) and facial features (posture, expression itself and the relationship with illumination), the performance of the model is evaluated by using frequency segmentation (FP), Laplacian pyramid (LP) and covariance crossover (CI) fusion techniques respectively. The model based on MDCT produces the results of PROM recognition. The recognition accuracy of chal time is 97.70, and the effectiveness and robustness are good.
- About the picture annotation of the manuscript. In order to make readers better distinguish between the text and notes, it is suggested that the font of the notes part of the manuscript is different from that of the text part, which can remind readers and make the boundary of the article clearer. Please refer the recent papers: Chenggang Yan, Biao Gong, Yuxuan Wei, Yue Gao, “Deep Multi-View Enhancement Hashing for Image Retrieval”, IEEE Transactions on Pattern Analysis and Machine Intelligence, 2020.Chenggang Yan, Zhisheng Li, Yongbing Zhang, Yutao Liu, Xiangyang Ji, Yongdong Zhang, “Depth image denoising using nuclear norm and learning graph model”, ACM Transactions on Multimedia Computing Communications and Applications 2020.Chenggang Yan, Yiming Hao, Liang Li, Jian Yin, Anan Liu, Zhendong Mao, Zhenyu Chen, Xingyu Gao, “Task-Adaptive Attention for Image Captioning”, IEEE Transactions on Circuits and Systems for Video Technology, 2021. Chenggang Yan, Tong Teng, Yutao Liu, Yongbing Zhang, Haoqian Wang, Xiangyang Ji, “Precise No-Reference Image Quality Evaluation Based on Distortion Identification”, ACM Transactions on Multimedia Computing Communications and Applications 2021.Chenggang Yan, Lixuan Meng, Liang Li, Jiehua Zhang, Jian Yin, Jiyong Zhang, Zhan Wang, Bolun Zheng, “Age-Invariant Face Recognition By Multi-Feature Fusion and Decomposition with Self-Attention”, ACM Transactions on Multimedia Computing Communications and Applications 2021
- Introduction to the manuscript. In order to make readers better understand the method proposed in the article and its advantages, it is suggested to quote some common examples in life when introducing the method in the introduction part, so as to make readers better understand the method proposed in the article.
- The manuscript tests the performance of the developed model in face recognition, including comparing and analyzing the accu classification performance of three advanced fusion level face recognition models, using FP, LP and CI fusion technology. Using MLP Ann condi condition, 10000 images were selected from ORL database and tested to evaluate the performance of models. The classification efficiency of the model reached the following levels: expression change (97.70%), illumination change (97.07%), low resolution (97.11%), occlusion (96.87%), and attitude change (96.66%).
- The research results of manuscripts show that the fusion level face recognition method has better application prospects than the face recognition method based on local and global features. Compared with CI, FP and LP fusion technology, the model studied has achieved higher accuracy on the whole.
Author Response
Reviewer:
The manuscript proposes a multi-resolution discrete cosine transform fusion technology, the unique fusion feature level face recognition model (fflfrm), including face detection, feature extraction, feature fusion and face classification.
According to the image features (low resolution problem and occlusion problem) and facial features (posture, expression itself and the relationship with illumination), the performance of the model is evaluated by using frequency segmentation (FP), Laplacian pyramid (LP) and covariance crossover (CI) fusion techniques respectively. The model based on MDCT produces the results of PROM recognition. The recognition accuracy of chal time is 97.70, and the effectiveness and robustness are good.
Answer: We thank the reviewer for this positive comment and clarification.
- About the picture annotation of the manuscript. In order to make readers better distinguish between the text and notes, it is suggested that the font of the notes part of the manuscript is different from that of the text part, which can remind readers and make the boundary of the article clearer.
Answer: The whole manuscript is carefully revised according to the journal format.
Please refer the recent papers: Chenggang Yan, Biao Gong, Yuxuan Wei, Yue Gao, “Deep Multi-View Enhancement Hashing for Image Retrieval”, IEEE Transactions on Pattern Analysis and Machine Intelligence, 2020.Chenggang Yan, Zhisheng Li, Yongbing Zhang, Yutao Liu, Xiangyang Ji, Yongdong Zhang, “Depth image denoising using nuclear norm and learning graph model”, ACM Transactions on Multimedia Computing Communications and Applications 2020.Chenggang Yan, Yiming Hao, Liang Li, Jian Yin, Anan Liu, Zhendong Mao, Zhenyu Chen, Xingyu Gao, “Task-Adaptive Attention for Image Captioning”, IEEE Transactions on Circuits and Systems for Video Technology, 2021. Chenggang Yan, Tong Teng, Yutao Liu, Yongbing Zhang, Haoqian Wang, Xiangyang Ji, “Precise No-Reference Image Quality Evaluation Based on Distortion Identification”, ACM Transactions on Multimedia Computing Communications and Applications 2021.Chenggang Yan, Lixuan Meng, Liang Li, Jiehua Zhang, Jian Yin, Jiyong Zhang, Zhan Wang, Bolun Zheng, “Age-Invariant Face Recognition By Multi-Feature Fusion and Decomposition with Self-Attention”, ACM Transactions on Multimedia Computing Communications and Applications 2021
Answer 1: the Yan et al. (2021) recent relevant related study have been added to the Literature Review section (Added in the Literature Review line 181).
“Yan et al. [57-61] suggested a Multi-feature Fusion and Decomposition (MFD) model based on the multi-head attention and the backbone network for age-invariant face recognition. The proposed model reduces the intra-class variants by learning the discriminative, efficient and robust features. CACD and CACD-VS databases were used for experimental investigations which demonstrated that the suggested model has better facial recognition accuracy than state-of-the-art models.”
Note: references of the new added relevant studies are re-numberd and added to the Refrences Section.
- Chenggang Yan, Biao Gong, Yuxuan Wei, Yue Gao, “Deep Multi-View Enhancement Hashing for Image Retrieval”, IEEE Transactions on Pattern Analysis and Machine Intelligence, 2020.
- Chenggang Yan, Zhisheng Li, Yongbing Zhang, Yutao Liu, Xiangyang Ji, Yongdong Zhang, “Depth image denoising using nuclear norm and learning graph model”, ACM Transactions on Multimedia Computing Communications and Applications 2020.
- Chenggang Yan, Yiming Hao, Liang Li, Jian Yin, Anan Liu, Zhendong Mao, Zhenyu Chen, Xingyu Gao, “Task-Adaptive Attention for Image Captioning”, IEEE Transactions on Circuits and Systems for Video Technology, 2021.
- Chenggang Yan, Tong Teng, Yutao Liu, Yongbing Zhang, Haoqian Wang, Xiangyang Ji, “Precise No-Reference Image Quality Evaluation Based on Distortion Identification”, ACM Transactions on Multimedia Computing Communications and Applications 2021.
- Chenggang Yan, Lixuan Meng, Liang Li, Jiehua Zhang, Jian Yin, Jiyong Zhang, Zhan Wang, Bolun Zheng, “Age-Invariant Face Recognition By Multi-Feature Fusion and Decomposition with Self-Attention”, ACM Transactions on Multimedia Computing Communications and Applications 2021
- Introduction to the manuscript. In order to make readers better understand the method proposed in the article and its advantages, it is suggested to quote some common examples in life when introducing the method in the introduction part, so as to make readers better understand the method proposed in the article.
Answer: The following paragraph is added to the introduction part, in order to explain the importance of the proposed model in our life (Added in the introduction line 87)
“The proposed MDCT fusion-based FLFRM has a significant impact on the real time face recognition applications, hence it able to analyze more sophisticated facial characteristics and to categorize images/ faces according to higher-order identity markets. In addition, it can be applied in many fields requiring identity verification and person location, such as finding missing children or criminals in crowds, and calling up customer or patient records from governmental or healthcare databases. “
- The manuscript tests the performance of the developed model in face recognition, including comparing and analyzing the accu classification performance of three advanced fusion level face recognition models, using FP, LP and CI fusion technology. Using MLP Ann condi condition, 10000 images were selected from ORL database and tested to evaluate the performance of models. The classification efficiency of the model reached the following levels: expression change (97.70%), illumination change (97.07%), low resolution (97.11%), occlusion (96.87%), and attitude change (96.66%).
Answer: We thank the reviewer for this positive comment and clarification.
- The research results of manuscripts show that the fusion level face recognition method has better application prospects than the face recognition method based on local and global features. Compared with CI, FP and LP fusion technology, the model studied has achieved higher accuracy on the whole.
Answer: We thank the reviewer for this positive comment and clarification.
Author Response File: Author Response.docx
Reviewer 2 Report
- The improvement of the proposed fusion method was not significant compared with three alternative approaches, i.e. Frequency Partition (FP), Laplacian Pyramid (LP) and Covariance Intersection (CI).
- Why does the authors choose MDCT for feature fusion? Could you provide further explanation? Since there are various transformation could be used, e.g. Discrete Fourier Transform, Discrete Wavelet Transform, etc.
- The proposed model is only tested on one dataset which is ORL. Could you provide more experiments on another large-scale dataset for face recognition?
Author Response
Reviewer # 2
- The improvement of the proposed fusion method was not significant compared with three alternative approaches, i.e. Frequency Partition (FP), Laplacian Pyramid (LP) and Covariance Intersection (CI).
Answer: We thank the reviewer for this positive comment and clarification.
- Why does the authors choose MDCT for feature fusion? Could you provide further explanation? Since there are various transformation could be used, e.g. Discrete Fourier Transform, Discrete Wavelet Transform, etc.
Answer: we have chosen the MDCT technique for features fusion among other transform techniques, due to its effectiveness in enhancing the fused images resolutions
Note: The following paragraph is added to the introduction part (Added in the introduction line 71)
“In this research, MDCT technique is selected for features fusion due to its effectiveness in enhancing the fused images resolutions [52].”
- The proposed model is only tested on one dataset which is ORL. Could you provide more experiments on another large-scale dataset for face recognition?
Answer1: ORL is benchmark and a large-scale dataset for face recognition, is used in this research due to it is publicity and availability , Furthermore it has been used by many researcher in this area such the work done by (Tran et al. [33], Nusir [38], Al-Shatnawi et al. [53], El-Bashir et al. [49] and many others ).
Answer 2: we added the following paragraph in the future work: “Furthermore, it will be interesting to test and evaluate the proposed model using another large-scale face recognition datasets"
Author Response File: Author Response.docx
Round 2
Reviewer 2 Report
Thank you the authors for their response.
The revised version does not provide additional experiment results to support the conclusion and reasoning for the choice of MDCT.
The authors only refer to another reference (2013) to explain their reasoning may not be enough.
Therefore, I come to my final decision of rejecting the paper as it needs additional experiments.
Author Response
Response Letter
Multi-Resolution Discrete Cosine Transform Fusion Technique Face Recognition Model
Bader M. AlFawwaz 1, Atallah AL-Shatnawi1, Faisal Al-Saqqar2 and Mohammad Nusir3
1* Information Systems Department, Prince Hussein Bin Abdullah College for Information Technology, Al al-Bayt University, Mafraq, Jordan
*Corresponding author: [email protected]; Tel.: (00962-6297000 Ext 3399)
2Computer Science Department, Prince Hussein Bin Abdullah College for Information Technology, Al al-Bayt University, Mafraq, Jordan
3CBM Integrated Software Inc (CBMIS), Amman, Jordan
First of all, we would like to thank and express our appreciation to the reviewer for taking the time to carefully review our manuscript, and for their insightful, valuable, and very useful comments. We would also like to thank the editor for his valuable comments and suggestions to improve this manuscript. We carefully considered the comments and implemented all of them in the revised version. In this letter, we will explain, point by point, how the issues raised in the reviewers’ reports are addressed and answered.
Reviewer # 2
- The improvement of the proposed fusion method was not significant compared with three alternative approaches, i.e. Frequency Partition (FP), Laplacian Pyramid (LP), and Covariance Intersection (CI).
Answer 1: The Experimental Results and Discussion section (Section 4) has been revised and improved by the following:
- The first and second paragraphs were revaised as the following (Line 283):
“The effective face recognition model has to ideally deal with a number of challenges. It mainly contains expression changes, illumination, images with low resolution, occlusion, and pose [2]. To validate the proposed FFLFRM based on MDCT model effectiveness, it has been compared with three state-of-the-art models that were developed based on FP [38], LP [53], and CI [49] features fusion techniques. The proposed FFLFRM based on MDCT technique and three state-of-the-art models were run using MATLAB@2015a programming language in a PC with Intel Core i7 processor (2.40 GHz, 8 GB RAM).
The proposed FFLFRM and three state-of-the-art models were tested and evaluated using MLP ANN for 10,000 face images derived from the ORL database based on the expression changes, illumination, and images with low resolution, occlusion, and pose challenges. The comparative evaluation results of their classification efficiencies are presented and summarized in Table 1, and are elaborated and discussed upon in more depth below. “
Table 1. Performance evaluation results of the proposed FFLFRM and the three state-of-the-art models classification efficiency.
|
Pose change |
Illumination change |
Expression change |
Low-resolution images |
Occlusion |
FRS based on FP fusion [38] |
97.02% |
96.47% |
97.73% |
96.99% |
96.18% |
FRS based on LP fusion [53] |
96.14% |
97.03% |
98.2% |
96.5% |
96.2% |
FRS based on CI fusion [49] |
96.23% |
96.89% |
97.68% |
96.1% |
96.84% |
FFLFRM based on MDCT |
96.66% |
97.07% |
97.70% |
97.11% |
96.87% |
- The “three comparator models” sentences has been changed in to “three state-of-the-art models ” in the whole manuscript.
- In order to prove the proposed based on MDCT model efficiency compared to the three state-of-the-art models ( i.e. Frequency Partition (FP), Laplacian Pyramid (LP), and Covariance Intersection (CI)), the following paragraph is added to the Experimental Results and Discussion section (line 359)
“Thus, based on the abovementioned five discussed challenges, the proposed FFLFRM based on MDCT model classification efficiency achieved the best results outperforming the other three state-of-the-art models in terms of illumination change, dealing with low-resolution images, and working effectively with occlusion-condition. But in terms of changing poses challenge, the proposed model achieved a proposing classification results that higher is than the two state-of-the-art based on LP and CI models. Furthermore, it achieved better classification efficiency compared to the CI state-of-the-art model under the condition of expression change. These results indicate the acceptability of the proposed FFLFRM effectiveness in facial recognition in the context of above mentioned challenges. Consequently, it proves the proposed FFLFRM based on MDCT model effectiveness compared to the three state-of-the-art models (i.e. Frequency Partition (FP), Laplacian Pyramid (LP), and Covariance Intersection (CI)).”
- Why do the authors choose MDCT for feature fusion? Could you provide a further explanation? Since various transformations could be used, e.g. Discrete Fourier Transform, Discrete Wavelet Transform, etc.
Answer 2: A complete experiment has been conducted, in order to explain why we have chosen MDCT for feature fusion among other transformation methods such as Discrete Fourier Transform (DFT), Fast Fourier Transform (FFT) and Discrete Wavelet Transform (DWT). The following paragraphs, Results and Figures are added in the Experimental Results and Discussion section (line 371 to 396)
“Moreover, to validate the proposed FFLFRM based on MDCT model effectiveness compared to various transformation methods such as Discrete Fourier Transform (DFT), Fast Fourier Transform (FFT) and Discrete Wavelet Transform (DWT), we have tested and evaluated the proposed FFLFRM and three abovementioned transformations methods using MLP ANN for 10,000 face images derived from the ORL database based on classification accuracy and exaction time during the training and testing phases. The comparative evaluation results of the proposed FFLFRM based on MDCT and the three abovementioned methods classification efficiencies are presented and summarized in Table 2, and graphically is shown in Figure 8. As well as, the executed time of the FFLFRM model using the four transformation methods (i.e. MDCT, DFT, FFT, and DW) during the training and testing phases using the ORL dataset are presented in Table 3.”
Table 2. Performance evaluation results of the proposed FFLFRM based on MDCT and the three transformation methods classification efficiency.
Methods |
MDCT |
DFT |
FFT |
DWT |
Recognition results |
97.7% |
96.8% |
96.3% |
97.5% |
Table 3. The proposed FFLFRM based on MDCT and the three transformation methods execution time on the ORL dataset.
Methods \Time |
MDCT |
DFT |
FFT |
DWT |
Training |
2.7 × 10 -5 |
1.2 × 10-4 |
2.7 × 10-4 |
1.9 ×10-2 |
Testing |
3.4 × 10-6 |
4.3 × 10-4 |
3.9 × 10-4 |
2.0010-3 |
“It is noticed that in table 2, the proposed FFLFRM model using MDCT method achieved the best classification accuracy outperforming the other three transformation methods (i.e. DFT, FFT, and DW) when tested on the ORL database using MLP ANN. Furthermore, Table 3 proves that, the proposed FFLFRM model using MDCT method is faster than the other three abovementioned transformation methods, and thus prove the effectiveness of the MDCT as feature fusion technique for fiscal real-life applications.”
Figure 7. Performance evaluation results of the proposed FFLFRM using MDCT, DFT, FFT, and DW fusion methods tested on the ORL database using MLP ANN.
- The proposed model is only tested on one dataset which is ORL. Could you provide more experiments on another large-scale dataset for face recognition?
Answer1: ORL is the benchmark and a large-scale dataset for face recognition, is used in this research due to it is publicity and availability, Furthermore, it has been used by many researchers in this area such as the work done by (Tran et al. [33], Nusir [38], Al-Shatnawi, et al. [53], El-Bashir et al. [49] and many others ).
Answer 2: we added the following paragraph in the future work: “Furthermore, it will be interesting to test and evaluate the proposed model using another large-scale face recognition dataset"
- We have done the following improvement as well:
- We have improved the conclusion and future works section by adding the following two paragraphs:
“The proposed FFLFRM based on MDCT model classification efficiency achieved the best results outperforming the other three state-of-the-art models in terms of illumination change, dealing with low-resolution images, and working effectively with occlusion-condition. In terms of dealing with low-resolution images, the proposed FFLFRM based on MDCT model produced (97.11%) classification accuracy, while the other three state-of-the-art models produced (96.99%, 96.5% and 96.1%) classification accuracies respectively. In terms of working effectively with occlusion the proposed FFLFRM based on MDCT model produced (96.87%) classification accuracy, while the other three state-of-the-art models produced (96.18%, 96.2% and 96.2%) classification accuracies respectively. In terms of illumination change the proposed FFLFRM based on MDCT model produced (97.07%) classification accuracy, while the other three state-of-the-art models produced (96.47%, 97.03%and 96.89%) classification accuracies respectively. By dealing with changing poses challenge, the proposed model achieved a proposing classification results that is higher than the two state-of-the-art based on LP and CI models. Hence it produced (96.66%) classification accuracy, while the other three state-of-the-art models produced (97.02%, 96.14% and 96.23%) classification accuracies respectively. Furthermore, it achieved better classification efficiency compared to the CI state-of-the-art model under the condition of expression change. Hence it produced (97.7%) classification accuracy, while the other three state-of-the-art models produced (97.73%, 98.2% and 97.68%) classification accuracies respectively. These results indicate the acceptability of the proposed FFLFRM effectiveness in facial recognition in the context of abovementioned challenges. Consequently, it proves the proposed FFLFRM based on MDCT model effectiveness compared to the three state-of-the-art models (i.e. Frequency Partition (FP), Laplacian Pyramid (LP), and Covariance Intersection (CI)).
Furthermore, the proposed FFLFRM based on MDCT model effectiveness is verified and compared with Discrete Fourier Transform (DFT), Fast Fourier Transform (FFT) and Discrete Wavelet Transform (DWT) transformation methods, in terms of classification accuracy and exaction time during the training and testing phases. The results proved that the proposed FFLFRM model using MDCT method achieved the best classification accuracy compared to the other three transformation methods when tested on the ORL database using MLP ANN. Hence, it produced (97.7%) classification accuracy where the other three methods produced (96.8%, 96.3% and 97.5%) classification accuracies respectively. The proposed FFLFRM based on MDCT model is faster than the other three transformation methods in both training and testing phases. Therefore, this paper concluded that, MDCT is simpler, faster and more accurate than the DFT, FFT, and DWT as well as, it is an effective method for facial real-life applications. “
- We have improved the abstract by adding the following paragraphs:
“Furthermore, this work proved that, the MDCT method used by the proposed FFLFRM is simpler, faster and more accurate than the Discrete Fourier Transform (DFT), Fast Fourier Transform (FFT) and Discrete Wavelet Transform (DWT) as well as, it is an effective method for facial real-life applications. ”
Author Response File: Author Response.docx
Round 3
Reviewer 2 Report
The revised version provides additional experiment results to support the conclusion and reasoning for the choice of MDCT. Thus, it is good enough for publishing after carefully checking for minor typos and grammatical error.
Author Response
Response Letter
Multi-Resolution Discrete Cosine Transform Fusion Technique Face Recognition Model
Bader M. AlFawwaz 1, Atallah AL-Shatnawi1, Faisal Al-Saqqar2 and Mohammad Nusir3
1* Information Systems Department, Prince Hussein Bin Abdullah College for Information Technology, Al al-Bayt University, Mafraq, Jordan
*Corresponding author: [email protected]; Tel.: (00962-6297000 Ext 3399)
2Computer Science Department, Prince Hussein Bin Abdullah College for Information Technology, Al al-Bayt University, Mafraq, Jordan
3CBM Integrated Software Inc (CBMIS), Amman, Jordan
First of all, we would like to thank and express our appreciation to the reviewers for taking the time to carefully review our manuscript, and for their insightful, valuable, and very useful comments. We would also like to thank the editor for his valuable comments and suggestions to improve this manuscript. In this paper, we have done the following.
- We have carefully revised the whole manuscript (Editing and proofreading).
- We have carefully formatted the whole manuscript according to the journal format.
- We have checked all the references in the whole manuscript.