Topic Menu
► Topic MenuTopic Editors
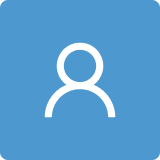
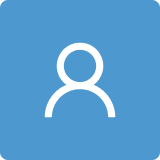
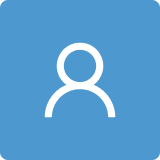
AI-enabled Sensor-related Technologies for Physiological Monitoring Designed to Advance Emergency Medical Care
Topic Information
Dear Colleagues,
Low circulating blood volume (hypovolemia) and airway obstruction resulting from life-threatening conditions such as trauma represent leading causes of compromised health and death globally in both civilian and military settings. A basic principle for effective treatment of any medical condition is that early detection and intervention will improve outcome. Most clinical guidelines for assessment of patient status are based on measurements of standard vital signs such as blood pressure and heart rate, which have proven to be neither sensitive in time nor specific to the individual patient or medical condition. The use of artificial intelligence (AI) incorporated into the development of new medical monitoring capabilities that integrate sensors with technologies that can expedite early detection of a clinical problem or guide the accuracy of interventions could prove critical to reducing morbidity and mortality, particularly in the prehospital setting where time is the most threatening element. The focus of this Special Issue is to provide descriptions of novel AI-enabled technologies designed to integrate sensors capable of providing the most sensitive and specific physiological measures combined with machine-learning algorithms that are currently being developed. The papers presented in this Topic will address strategies that can be used to advance medical decision support during the critical early stages of emergency care.
Prof. Dr. Victor A Convertino
Prof. Dr. Omer T Inan
Dr. Sylvain Cardin
Topic Editors
Keywords
- hemorrhage
- physiological monitoring
- machine learning
- biofeedback
- decision support
- goal-directed resuscitation
Participating Journals
Journal Name | Impact Factor | CiteScore | Launched Year | First Decision (median) | APC |
---|---|---|---|---|---|
![]()
Biosensors
|
4.9 | 6.6 | 2011 | 17.1 Days | CHF 2700 |
![]()
Sensors
|
3.4 | 7.3 | 2001 | 16.8 Days | CHF 2600 |
MDPI Topics is cooperating with Preprints.org and has built a direct connection between MDPI journals and Preprints.org. Authors are encouraged to enjoy the benefits by posting a preprint at Preprints.org prior to publication:
- Immediately share your ideas ahead of publication and establish your research priority;
- Protect your idea from being stolen with this time-stamped preprint article;
- Enhance the exposure and impact of your research;
- Receive feedback from your peers in advance;
- Have it indexed in Web of Science (Preprint Citation Index), Google Scholar, Crossref, SHARE, PrePubMed, Scilit and Europe PMC.