-
Optimal Feature Selection and Classification for Parkinson’s Disease Using Deep Learning and Dynamic Bag of Features Optimization
-
A Network Analysis Approach to Detect and Differentiate Usher Syndrome Types Using miRNA Expression Profiles: A Pilot Study
-
Quantifying Lenition as a Diagnostic Marker for Parkinson’s Disease and Atypical Parkinsonism
-
Implementation of Automatic Segmentation Framework as Preprocessing Step for Radiomics Analysis of Lung Anatomical Districts
Journal Description
BioMedInformatics
BioMedInformatics
is an international, peer-reviewed, open access journal on all areas of biomedical informatics, as well as computational biology and medicine, published quarterly online by MDPI.
- Open Access— free for readers, with article processing charges (APC) paid by authors or their institutions.
- High Visibility: indexed within Scopus, EBSCO, and other databases.
- Rapid Publication: manuscripts are peer-reviewed and a first decision is provided to authors approximately 22 days after submission; acceptance to publication is undertaken in 6.2 days (median values for papers published in this journal in the second half of 2024).
- Journal Rank: CiteScore - Q2 (Health Professions (miscellaneous))
- Recognition of Reviewers: APC discount vouchers, optional signed peer review, and reviewer names published annually in the journal.
Latest Articles
Time–Frequency Transformations for Enhanced Biomedical Signal Classification with Convolutional Neural Networks
BioMedInformatics 2025, 5(1), 7; https://doi.org/10.3390/biomedinformatics5010007 - 27 Jan 2025
Abstract
►
Show Figures
Background: Transforming one-dimensional (1D) biomedical signals into two-dimensional (2D) images enables the application of convolutional neural networks (CNNs) for classification tasks. In this study, we investigated the effectiveness of different 1D-to-2D transformation methods to classify electrocardiogram (ECG) and electroencephalogram (EEG) signals. Methods: We
[...] Read more.
Background: Transforming one-dimensional (1D) biomedical signals into two-dimensional (2D) images enables the application of convolutional neural networks (CNNs) for classification tasks. In this study, we investigated the effectiveness of different 1D-to-2D transformation methods to classify electrocardiogram (ECG) and electroencephalogram (EEG) signals. Methods: We select five transformation methods: Continuous Wavelet Transform (CWT), Fast Fourier Transform (FFT), Short-Time Fourier Transform (STFT), Signal Reshaping (SR), and Recurrence Plots (RPs). We used the MIT-BIH Arrhythmia Database for ECG signals and the Epilepsy EEG Dataset from the University of Bonn for EEG signals. After converting the signals from 1D to 2D, using the aforementioned methods, we employed two types of 2D CNNs: a minimal CNN and the LeNet-5 model. Our results indicate that RPs, CWT, and STFT are the methods to achieve the highest accuracy across both CNN architectures. Results: These top-performing methods achieved accuracies of 99%, 98%, and 95%, respectively, on the minimal 2D CNN and accuracies of 99%, 99%, and 99%, respectively, on the LeNet-5 model for the ECG signals. For the EEG signals, all three methods achieved accuracies of 100% on the minimal 2D CNN and accuracies of 100%, 99%, and 99% on the LeNet-5 2D CNN model, respectively. Conclusions: This superior performance is most likely related to the methods’ capacity to capture time–frequency information and nonlinear dynamics inherent in time-dependent signals such as ECGs and EEGs. These findings underline the significance of using appropriate transformation methods, suggesting that the incorporation of time–frequency analysis and nonlinear feature extraction in the transformation process improves the effectiveness of CNN-based classification for biological data.
Full article
Open AccessArticle
Hybrid Neural Network Models to Estimate Vital Signs from Facial Videos
by
Yufeng Zheng
BioMedInformatics 2025, 5(1), 6; https://doi.org/10.3390/biomedinformatics5010006 - 22 Jan 2025
Abstract
Introduction: Remote health monitoring plays a crucial role in telehealth services and the effective management of patients, which can be enhanced by vital sign prediction from facial videos. Facial videos are easily captured through various imaging devices like phone cameras, webcams, or
[...] Read more.
Introduction: Remote health monitoring plays a crucial role in telehealth services and the effective management of patients, which can be enhanced by vital sign prediction from facial videos. Facial videos are easily captured through various imaging devices like phone cameras, webcams, or surveillance systems. Methods: This study introduces a hybrid deep learning model aimed at estimating heart rate (HR), blood oxygen saturation level (SpO2), and blood pressure (BP) from facial videos. The hybrid model integrates convolutional neural network (CNN), convolutional long short-term memory (convLSTM), and video vision transformer (ViViT) architectures to ensure comprehensive analysis. Given the temporal variability of HR and BP, emphasis is placed on temporal resolution during feature extraction. The CNN processes video frames one by one while convLSTM and ViViT handle sequences of frames. These high-resolution temporal features are fused to predict HR, BP, and SpO2, capturing their dynamic variations effectively. Results: The dataset encompasses 891 subjects of diverse races and ages, and preprocessing includes facial detection and data normalization. Experimental results demonstrate high accuracies in predicting HR, SpO2, and BP using the proposed hybrid models. Discussion: Facial images can be easily captured using smartphones, which offers an economical and convenient solution for vital sign monitoring, particularly beneficial for elderly individuals or during outbreaks of contagious diseases like COVID-19. The proposed models were only validated on one dataset. However, the dataset (size, representation, diversity, balance, and processing) plays an important role in any data-driven models including ours. Conclusions: Through experiments, we observed the hybrid model’s efficacy in predicting vital signs such as HR, SpO2, SBP, and DBP, along with demographic variables like sex and age. There is potential for extending the hybrid model to estimate additional vital signs such as body temperature and respiration rate.
Full article
(This article belongs to the Section Applied Biomedical Data Science)
►▼
Show Figures
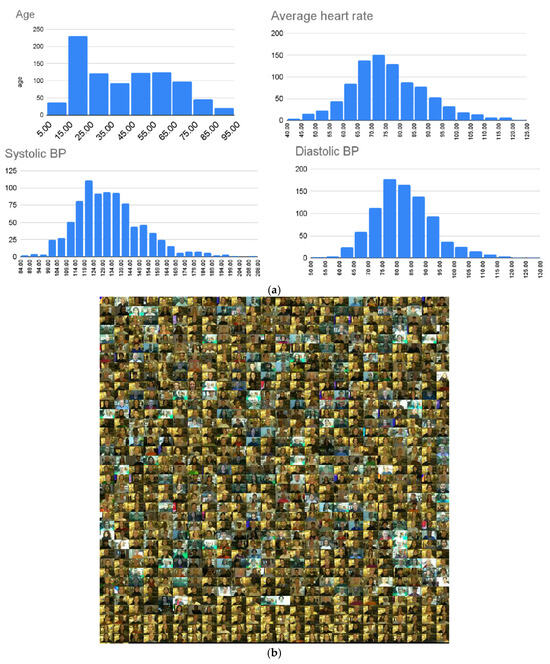
Figure 1
Open AccessArticle
Advancing Emotion Recognition: EEG Analysis and Machine Learning for Biomedical Human–Machine Interaction
by
Sara Reis, Luís Pinto-Coelho, Maria Sousa, Mariana Neto and Marta Silva
BioMedInformatics 2025, 5(1), 5; https://doi.org/10.3390/biomedinformatics5010005 - 10 Jan 2025
Abstract
►▼
Show Figures
Background: Human emotions are subjective psychophysiological processes that play an important role in the daily interactions of human life. Emotions often do not manifest themselves in isolation; people can experience a mixture of them and may not express them in a visible or
[...] Read more.
Background: Human emotions are subjective psychophysiological processes that play an important role in the daily interactions of human life. Emotions often do not manifest themselves in isolation; people can experience a mixture of them and may not express them in a visible or perceptible way; Methods: This study seeks to uncover EEG patterns linked to emotions, as well as to examine brain activity across emotional states and optimise machine learning techniques for accurate emotion classification. For these purposes, the DEAP dataset was used to comprehensively analyse electroencephalogram (EEG) data and understand how emotional patterns can be observed. Machine learning algorithms, such as SVM, MLP, and RF, were implemented to predict valence and arousal classifications for different combinations of frequency bands and brain regions; Results: The analysis reaffirms the value of EEG as a tool for objective emotion detection, demonstrating its potential in both clinical and technological contexts. By highlighting the benefits of using fewer electrodes, this study emphasises the feasibility of creating more accessible and user-friendly emotion recognition systems; Conclusions: Further improvements in feature extraction and model generalisation are necessary for clinical applications. This study highlights not only the potential of emotion classification to develop biomedical applications, but also to enhance human–machine interaction systems.
Full article
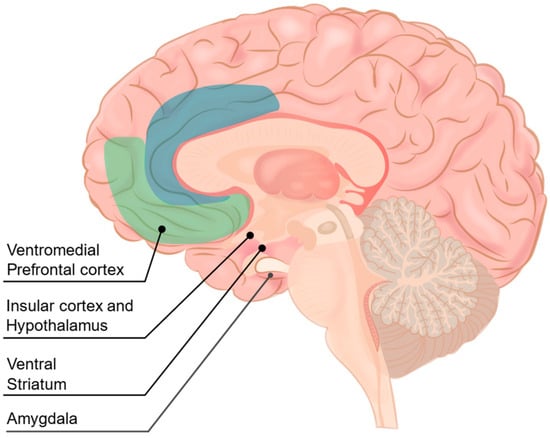
Figure 1
Open AccessArticle
Validation of an Upgraded Virtual Reality Platform Designed for Real-Time Dialogical Psychotherapies
by
Taylor Simoes-Gomes, Stéphane Potvin, Sabrina Giguère, Mélissa Beaudoin, Kingsada Phraxayavong and Alexandre Dumais
BioMedInformatics 2025, 5(1), 4; https://doi.org/10.3390/biomedinformatics5010004 - 9 Jan 2025
Abstract
►▼
Show Figures
Background: The advent of virtual reality in psychiatry presents a wealth of opportunities for a variety of psychopathologies. Avatar Interventions are dialogic and experiential treatments integrating personalized medicine with virtual reality (VR), which have shown promising results by enhancing the emotional regulation of
[...] Read more.
Background: The advent of virtual reality in psychiatry presents a wealth of opportunities for a variety of psychopathologies. Avatar Interventions are dialogic and experiential treatments integrating personalized medicine with virtual reality (VR), which have shown promising results by enhancing the emotional regulation of their participants. Notably, Avatar Therapy for the treatment of auditory hallucinations (i.e., voices) allows patients to engage in dialogue with an avatar representing their most persecutory voice. In addition, Avatar Intervention for cannabis use disorder involves an avatar representing a significant person in the patient’s consumption. In both cases, the main goal is to modify the problematic relationship and allow patients to regain control over their symptoms. While results are promising, its potential to be applied to other psychopathologies, such as major depression, is an exciting area for further exploration. In an era where VR interventions are gaining popularity, the present study aims to investigate whether technological advancements could overcome current limitations, such as avatar realism, and foster a deeper immersion into virtual environments, thereby enhancing participants’ sense of presence within the virtual world. A newly developed virtual reality platform was compared to the current platform used by our research team in past and ongoing studies. Methods: This study involved 43 subjects: 20 healthy subjects and 23 subjects diagnosed with severe mental disorders. Each participant interacted with an avatar using both platforms. After each immersive session, questionnaires were administered by a graduate student in a double-blind manner to evaluate technological advancements and user experiences. Results: The findings indicate that the new technological improvements allow the new platform to significantly surpass the current platform as per multiple subjective parameters. Notably, the new platform was associated with superior realism of the avatar (d = 0.574; p < 0.001) and the voice (d = 1.035; p < 0.001), as well as enhanced lip synchronization (d = 0.693; p < 0.001). Participants reported a significantly heightened sense of presence (d = 0.520; p = 0.002) and an overall better immersive experience (d = 0.756; p < 0.001) with the new VR platform. These observations were true in both healthy subjects and participants with severe mental disorders. Conclusions: The technological improvements generated a heightened sense of presence among participants, thus improving their immersive experience. These two parameters could be associated with the effectiveness of VR interventions and future studies should be undertaken to evaluate their impact on outcomes.
Full article
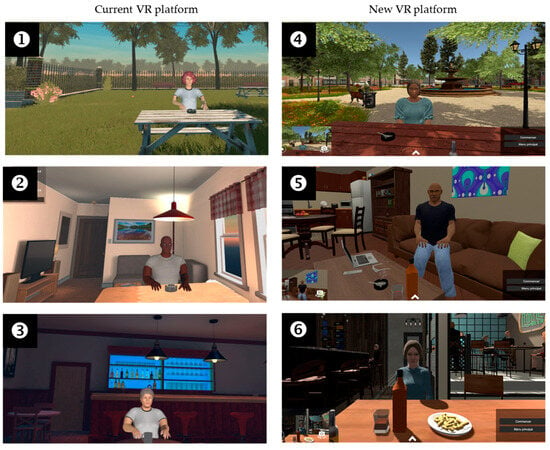
Figure 1
Open AccessArticle
Machine-Learning-Based Biomechanical Feature Analysis for Orthopedic Patient Classification with Disc Hernia and Spondylolisthesis
by
Daniel Nasef, Demarcus Nasef, Viola Sawiris, Peter Girgis and Milan Toma
BioMedInformatics 2025, 5(1), 3; https://doi.org/10.3390/biomedinformatics5010003 - 7 Jan 2025
Abstract
►▼
Show Figures
(1) Background: The exploration of various machine learning (ML) algorithms for classifying the state of Lumbar Intervertebral Discs (IVD) in orthopedic patients is the focus of this study. The classification is based on six key biomechanical features of the pelvis and lumbar
[...] Read more.
(1) Background: The exploration of various machine learning (ML) algorithms for classifying the state of Lumbar Intervertebral Discs (IVD) in orthopedic patients is the focus of this study. The classification is based on six key biomechanical features of the pelvis and lumbar spine. Although previous research has demonstrated the effectiveness of ML models in diagnosing IVD pathology using imaging modalities, there is a scarcity of studies using biomechanical features. (2) Methods: The study utilizes a dataset that encompasses two classification tasks. The first task classifies patients into Normal and Abnormal based on their IVDs (2C). The second task further classifies patients into three groups: Normal, Disc Hernia, and Spondylolisthesis (3C). The performance of various ML models, including decision trees, support vector machines, and neural networks, is evaluated using metrics such as accuracy, AUC, recall, precision, F1, Kappa, and MCC. These models are trained on two open-source datasets, using the PyCaret library in Python. (3) Results: The findings suggest that an ensemble of Random Forest and Logistic Regression models performs best for the 2C classification, while the Extra Trees classifier performs best for the 3C classification. The models demonstrate an accuracy of up to 90.83% and a precision of up to 91.86%, highlighting the effectiveness of ML models in diagnosing IVD pathology. The analysis of the weight of different biomechanical features in the decision-making processes of the models provides insights into the biomechanical changes involved in the pathogenesis of Lumbar IVD abnormalities. (4) Conclusions: This research contributes to the ongoing efforts to leverage data-driven ML models in improving patient outcomes in orthopedic care. The effectiveness of the models for both diagnosis and furthering understanding of Lumbar IVD herniations and spondylolisthesis is outlined. The limitations of AI use in clinical settings are discussed, and areas for future improvement to create more accurate and informative models are suggested.
Full article
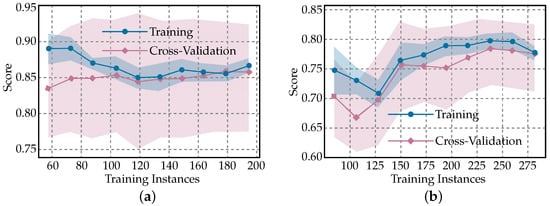
Figure 1
Open AccessArticle
A Data-Driven Approach to Revolutionize Children’s Vaccination with the Use of VR and a Novel Vaccination Protocol
by
Stavros Antonopoulos, Manolis Wallace and Vassilis Poulopoulos
BioMedInformatics 2025, 5(1), 2; https://doi.org/10.3390/biomedinformatics5010002 - 30 Dec 2024
Abstract
Background: This study aims to revolutionize traditional pediatric vaccination protocols by integrating virtual reality (VR) technology. The purpose is to minimize discomfort in children, ages 2–12, during vaccinations by immersing them in a specially designed VR short story that aligns with the various
[...] Read more.
Background: This study aims to revolutionize traditional pediatric vaccination protocols by integrating virtual reality (VR) technology. The purpose is to minimize discomfort in children, ages 2–12, during vaccinations by immersing them in a specially designed VR short story that aligns with the various stages of the clinical vaccination process. In our approach, the child dons a headset during the vaccination procedure and engages with a virtual reality (VR) short story that is specifically designed to correspond with the stages of a typical vaccination process in a clinical setting. Methods: A two-phase clinical trial was conducted to evaluate the effectiveness of the VR intervention. The first phase included 242 children vaccinated without VR, serving as a control group, while the second phase involved 97 children who experienced VR during vaccination. Discomfort levels were measured using the VACS (VAccination disComfort Scale) tool. Statistical analyses were performed to compare discomfort levels based on age, phases of vaccination, and overall experience. Results: The findings revealed significant reductions in discomfort among children who experienced VR compared to those in the control group. The VR intervention demonstrated superiority across multiple dimensions, including age stratification and different stages of the vaccination process. Conclusions: The proposed VR framework significantly reduces vaccination-related discomfort in children. Its cost-effectiveness, utilizing standard or low-cost headsets like Cardboard devices, makes it a feasible and innovative solution for pediatric practices. This approach introduces a novel, child-centric enhancement to vaccination protocols, improving the overall experience for young patients.
Full article
(This article belongs to the Section Clinical Informatics)
►▼
Show Figures
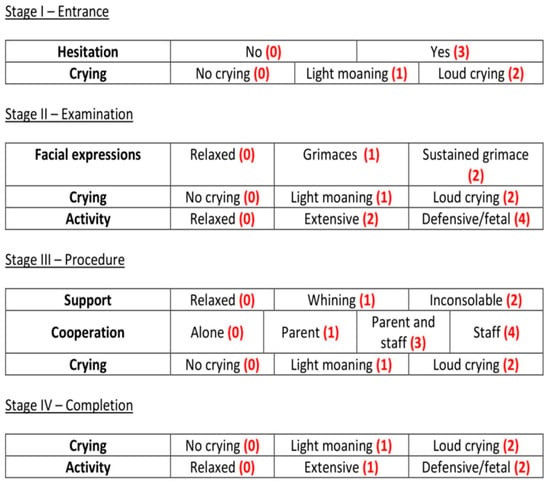
Figure 1
Open AccessArticle
Explainable Machine Learning-Based Approach to Identify People at Risk of Diabetes Using Physical Activity Monitoring
by
Simon Lebech Cichosz, Clara Bender and Ole Hejlesen
BioMedInformatics 2025, 5(1), 1; https://doi.org/10.3390/biomedinformatics5010001 - 24 Dec 2024
Abstract
►▼
Show Figures
Objective: This study aimed to investigate the utilization of patterns derived from physical activity monitoring (PAM) for the identification of individuals at risk of type 2 diabetes mellitus (T2DM) through an at-home screening approach employing machine learning techniques. Methods: Data from the 2011–2014
[...] Read more.
Objective: This study aimed to investigate the utilization of patterns derived from physical activity monitoring (PAM) for the identification of individuals at risk of type 2 diabetes mellitus (T2DM) through an at-home screening approach employing machine learning techniques. Methods: Data from the 2011–2014 National Health and Nutrition Examination Survey (NHANES) were scrutinized, focusing on the PAM component. The primary objective involved the identification of diabetes, characterized by an HbA1c ≥ 6.5% (48 mmol/mol), while the secondary objective included individuals with prediabetes, defined by an HbA1c ≥ 5.7% (39 mmol/mol). Features derived from PAM, along with age, were utilized as inputs for an XGBoost classification model. SHapley Additive exPlanations (SHAP) was employed to enhance the interpretability of the models. Results: The study included 7532 subjects with both PAM and HbA1c data. The model, which solely included PAM features, had a test dataset ROC-AUC of 0.74 (95% CI = 0.72–0.76). When integrating the PAM features with age, the model’s ROC-AUC increased to 0.79 (95% CI = 0.78–0.80) in the test dataset. When addressing the secondary target of prediabetes, the XGBoost model exhibited a test dataset ROC-AUC of 0.80 [95% CI; 0.79–0.81]. Conclusions: The objective quantification of physical activity through PAM yields valuable information that can be employed in the identification of individuals with undiagnosed diabetes and prediabetes.
Full article
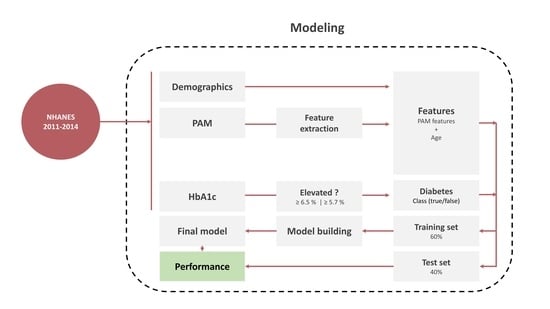
Graphical abstract
Open AccessArticle
Uncovering the Diagnostic Power of Radiomic Feature Significance in Automated Lung Cancer Detection: An Integrative Analysis of Texture, Shape, and Intensity Contributions
by
Sotiris Raptis, Christos Ilioudis and Kiki Theodorou
BioMedInformatics 2024, 4(4), 2400-2425; https://doi.org/10.3390/biomedinformatics4040129 - 18 Dec 2024
Abstract
►▼
Show Figures
Background: Lung cancer still maintains the leading position among causes of death in the world; the process of early detection surely contributes to changes in the survival of patients. Standard diagnostic methods are grossly insensitive, especially in the early stages. In this paper,
[...] Read more.
Background: Lung cancer still maintains the leading position among causes of death in the world; the process of early detection surely contributes to changes in the survival of patients. Standard diagnostic methods are grossly insensitive, especially in the early stages. In this paper, radiomic features are discussed that can assure improved diagnostic accuracy through automated lung cancer detection by considering the important feature categories, such as texture, shape, and intensity, originating from the CT DICOM images. Methods: We developed and compared the performance of two machine learning models—DenseNet-201 CNN and XGBoost—trained on radiomic features with the ability to identify malignant tumors from benign ones. Feature importance was analyzed using SHAP and techniques of permutation importance that enhance both the global and case-specific interpretability of the models. Results: A few features that reflect tumor heterogeneity and morphology include GLCM Entropy, shape compactness, and surface-area-to-volume ratio. These performed excellently in diagnosis, with DenseNet-201 producing an accuracy of 92.4% and XGBoost at 89.7%. The analysis of feature interpretability ascertains its potential in early detection and boosting diagnostic confidence. Conclusions: The current work identifies the most important radiomic features and quantifies their diagnostic significance through a properly conducted feature selection process reflecting stability analysis. This provides the blueprint for feature-driven model interpretability in clinical applications. Radiomics features have great value in the automated diagnosis of lung cancer, especially when combined with machine learning models. This might improve early detection and open personalized diagnostic strategies for precision oncology.
Full article
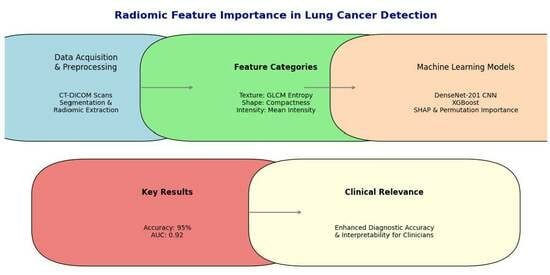
Graphical abstract
Open AccessArticle
Analysis of Regions of Homozygosity: Revisited Through New Bioinformatic Approaches
by
Susana Valente, Mariana Ribeiro, Jennifer Schnur, Filipe Alves, Nuno Moniz, Dominik Seelow, João Parente Freixo, Paulo Filipe Silva and Jorge Oliveira
BioMedInformatics 2024, 4(4), 2374-2399; https://doi.org/10.3390/biomedinformatics4040128 - 16 Dec 2024
Abstract
►▼
Show Figures
Background: Runs of homozygosity (ROHs), continuous homozygous regions across the genome, are often linked to consanguinity, with their size and frequency reflecting shared parental ancestry. Homozygosity mapping (HM) leverages ROHs to identify genes associated with autosomal recessive diseases. Whole-exome sequencing (WES) improves
[...] Read more.
Background: Runs of homozygosity (ROHs), continuous homozygous regions across the genome, are often linked to consanguinity, with their size and frequency reflecting shared parental ancestry. Homozygosity mapping (HM) leverages ROHs to identify genes associated with autosomal recessive diseases. Whole-exome sequencing (WES) improves HM by detecting ROHs and disease-causing variants. Methods: To streamline personalized multigene panel creation, using WES and ROHs, we developed a methodology integrating ROHMMCLI and HomozygosityMapper algorithms, and, optionally, Human Phenotype Ontology (HPO) terms, implemented in a Django Web application. Resorting to a dataset of 12,167 WES, we performed the first ROH profiling of the Portuguese population. Clustering models were applied to predict consanguinity from ROH features. Results: These resources were applied for the genetic characterization of two siblings with epilepsy, myoclonus and dystonia, pinpointing the CSTB gene as disease-causing. Using the 2021 Census population distribution, we created a representative sample (3941 WES) and measured genome-wide autozygosity (FROH). Portalegre, Viseu, Bragança, Madeira, and Vila Real districts presented the highest FROH scores. Multidimensional scaling showed that ROH count and sum were key predictors of consanguinity, achieving a test F1-score of 0.96 with additional features. Conclusions: This study contributes with new bioinformatics tools for ROH analysis in a clinical setting, providing unprecedented population-level ROH data for Portugal.
Full article
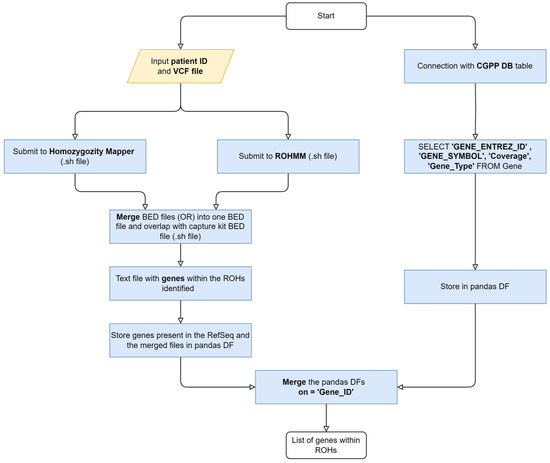
Figure 1
Open AccessArticle
Early Breast Cancer Detection Based on Deep Learning: An Ensemble Approach Applied to Mammograms
by
Youness Khourdifi, Alae El Alami, Mounia Zaydi, Yassine Maleh and Omar Er-Remyly
BioMedInformatics 2024, 4(4), 2338-2373; https://doi.org/10.3390/biomedinformatics4040127 - 13 Dec 2024
Abstract
Background: Breast cancer is one of the leading causes of death in women, making early detection through mammography crucial for improving survival rates. However, human interpretation of mammograms is often prone to diagnostic errors. This study addresses the challenge of improving the
[...] Read more.
Background: Breast cancer is one of the leading causes of death in women, making early detection through mammography crucial for improving survival rates. However, human interpretation of mammograms is often prone to diagnostic errors. This study addresses the challenge of improving the accuracy of breast cancer detection by leveraging advanced machine learning techniques. Methods: We propose an extended ensemble deep learning model that integrates three state-of-the-art convolutional neural network (CNN) architectures: VGG16, DenseNet121, and InceptionV3. The model utilizes multi-scale feature extraction to enhance the detection of both benign and malignant masses in mammograms. This ensemble approach is evaluated on two benchmark datasets: INbreast and CBIS-DDSM. Results: The proposed ensemble model achieved significant performance improvements. On the INbreast dataset, the ensemble model attained an accuracy of 90.1%, recall of 88.3%, and an F1-score of 89.1%. For the CBIS-DDSM dataset, the model reached 89.5% accuracy and 90.2% specificity. The ensemble method outperformed each individual CNN model, reducing both false positives and false negatives, thereby providing more reliable diagnostic results. Conclusions: The ensemble deep learning model demonstrated strong potential as a decision support tool for radiologists, offering more accurate and earlier detection of breast cancer. By leveraging the complementary strengths of multiple CNN architectures, this approach can improve clinical decision making and enhance the accessibility of high-quality breast cancer screening.
Full article
(This article belongs to the Topic Computational Intelligence and Bioinformatics (CIB))
►▼
Show Figures
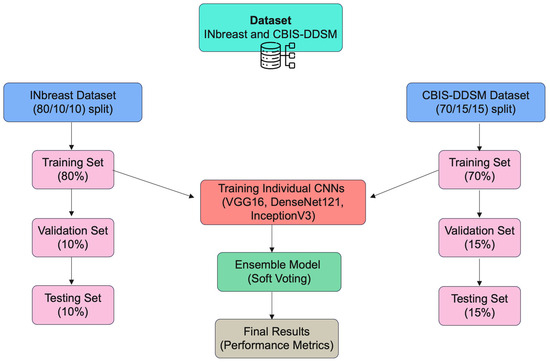
Figure 1
Open AccessReview
Artificial Intelligence in Wound Care: A Narrative Review of the Currently Available Mobile Apps for Automatic Ulcer Segmentation
by
Davide Griffa, Alessio Natale, Yuri Merli, Michela Starace, Nico Curti, Martina Mussi, Gastone Castellani, Davide Melandri, Bianca Maria Piraccini and Corrado Zengarini
BioMedInformatics 2024, 4(4), 2321-2337; https://doi.org/10.3390/biomedinformatics4040126 - 11 Dec 2024
Abstract
Introduction: Chronic ulcers significantly burden healthcare systems, requiring precise measurement and assessment for effective treatment. Traditional methods, such as manual segmentation, are time-consuming and error-prone. This review evaluates the potential of artificial intelligence AI-powered mobile apps for automated ulcer segmentation and their application
[...] Read more.
Introduction: Chronic ulcers significantly burden healthcare systems, requiring precise measurement and assessment for effective treatment. Traditional methods, such as manual segmentation, are time-consuming and error-prone. This review evaluates the potential of artificial intelligence AI-powered mobile apps for automated ulcer segmentation and their application in clinical settings. Methods: A comprehensive literature search was conducted across PubMed, CINAHL, Cochrane, and Google Scholar databases. The review focused on mobile apps that use fully automatic AI algorithms for wound segmentation. Apps requiring additional hardware or needing more technical documentation were excluded. Vital technological features, clinical validation, and usability were analysed. Results: Ten mobile apps were identified, showing varying levels of segmentation accuracy and clinical validation. However, many apps did not publish sufficient information on the segmentation methods or algorithms used, and most lacked details on the databases employed for training their AI models. Additionally, several apps were unavailable in public repositories, limiting their accessibility and independent evaluation. These factors challenge their integration into clinical practice despite promising preliminary results. Discussion: AI-powered mobile apps offer significant potential for improving wound care by enhancing diagnostic accuracy and reducing the burden on healthcare professionals. Nonetheless, the lack of transparency regarding segmentation techniques, unpublished databases, and the limited availability of many apps in public repositories remain substantial barriers to widespread clinical adoption. Conclusions: AI-driven mobile apps for ulcer segmentation could revolutionise chronic wound management. However, overcoming limitations related to transparency, data availability, and accessibility is essential for their successful integration into healthcare systems.
Full article
(This article belongs to the Section Imaging Informatics)
►▼
Show Figures
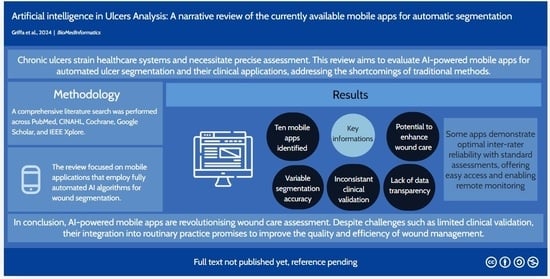
Graphical abstract
Open AccessArticle
Implementation of Automatic Segmentation Framework as Preprocessing Step for Radiomics Analysis of Lung Anatomical Districts
by
Alessandro Stefano, Fabiano Bini, Nicolò Lauciello, Giovanni Pasini, Franco Marinozzi and Giorgio Russo
BioMedInformatics 2024, 4(4), 2309-2320; https://doi.org/10.3390/biomedinformatics4040125 - 11 Dec 2024
Abstract
Background: The advent of artificial intelligence has significantly impacted radiology, with radiomics emerging as a transformative approach that extracts quantitative data from medical images to improve diagnostic and therapeutic accuracy. This study aimed to enhance the radiomic workflow by applying deep learning, through
[...] Read more.
Background: The advent of artificial intelligence has significantly impacted radiology, with radiomics emerging as a transformative approach that extracts quantitative data from medical images to improve diagnostic and therapeutic accuracy. This study aimed to enhance the radiomic workflow by applying deep learning, through transfer learning, for the automatic segmentation of lung regions in computed tomography scans as a preprocessing step. Methods: Leveraging a pipeline articulated in (i) patient-based data splitting, (ii) intensity normalization, (iii) voxel resampling, (iv) bed removal, (v) contrast enhancement and (vi) model training, a DeepLabV3+ convolutional neural network (CNN) was fine tuned to perform whole-lung-region segmentation. Results: The trained model achieved high accuracy, Dice coefficient (0.97) and BF (93.06%) scores, and it effectively preserved lung region areas and removed confounding anatomical regions such as the heart and the spine. Conclusions: This study introduces a deep learning framework for the automatic segmentation of lung regions in CT images, leveraging an articulated pipeline and demonstrating excellent performance of the model, effectively isolating lung regions while excluding confounding anatomical structures. Ultimately, this work paves the way for more efficient, automated preprocessing tools in lung cancer detection, with potential to significantly improve clinical decision making and patient outcomes.
Full article
(This article belongs to the Section Imaging Informatics)
►▼
Show Figures
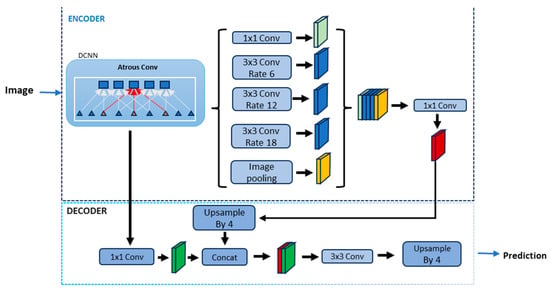
Figure 1
Open AccessEditorial
Should We Expect a Second Wave of AlphaFold Misuse After the Nobel Prize?
by
Alexandre G. de Brevern
BioMedInformatics 2024, 4(4), 2306-2308; https://doi.org/10.3390/biomedinformatics4040124 - 6 Dec 2024
Abstract
AlphaFold (AF) was the first deep learning tool to achieve exceptional fame in the field of biology [...]
Full article
Open AccessArticle
Quantifying Lenition as a Diagnostic Marker for Parkinson’s Disease and Atypical Parkinsonism
by
Ratree Wayland, Rachel Meyer, Ruhi Reddy, Kevin Tang and Karen W. Hegland
BioMedInformatics 2024, 4(4), 2287-2305; https://doi.org/10.3390/biomedinformatics4040123 - 29 Nov 2024
Abstract
►▼
Show Figures
Objective: This study aimed to evaluate lenition, a phonological process involving consonant weakening, as a diagnostic marker for differentiating Parkinson’s Disease (PD) from Atypical Parkinsonism (APD). Early diagnosis is critical for optimizing treatment outcomes, and lenition patterns in stop consonants may provide valuable
[...] Read more.
Objective: This study aimed to evaluate lenition, a phonological process involving consonant weakening, as a diagnostic marker for differentiating Parkinson’s Disease (PD) from Atypical Parkinsonism (APD). Early diagnosis is critical for optimizing treatment outcomes, and lenition patterns in stop consonants may provide valuable insights into the distinct motor speech impairments associated with these conditions. Methods: Using Phonet, a machine learning model trained to detect phonological features, we analyzed the posterior probabilities of continuant and sonorant features from the speech of 142 participants (108 PD, 34 APD). Lenition was quantified based on deviations from expected values, and linear mixed-effects models were applied to compare phonological patterns between the two groups. Results: PD patients exhibited more stable articulatory patterns, particularly in preserving the contrast between voiced and voiceless stops. In contrast, APD patients showed greater lenition, particularly in voiceless stops, coupled with increased articulatory variability, reflecting a more generalized motor deficit. Conclusions: Lenition patterns, especially in voiceless stops, may serve as non-invasive markers for distinguishing PD from APD. These findings suggest potential applications in early diagnosis and tracking disease progression. Future research should expand the analysis to include a broader range of phonological features and contexts to improve diagnostic accuracy.
Full article
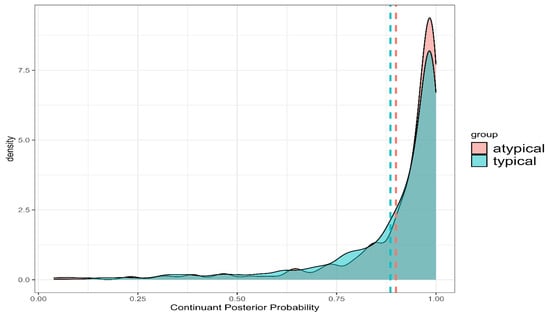
Figure 1
Open AccessArticle
A Network Analysis Approach to Detect and Differentiate Usher Syndrome Types Using miRNA Expression Profiles: A Pilot Study
by
Rama Krishna Thelagathoti, Wesley A. Tom, Chao Jiang, Dinesh S. Chandel, Gary Krzyzanowski, Appolinaire Olou and Rohan M. Fernando
BioMedInformatics 2024, 4(4), 2271-2286; https://doi.org/10.3390/biomedinformatics4040122 - 26 Nov 2024
Abstract
►▼
Show Figures
Background: Usher syndrome (USH) is a rare genetic disorder that affects both hearing and vision. It presents in three clinical types—USH1, USH2, and USH3—with varying onset, severity, and disease progression. Existing diagnostics primarily rely on genetic profiling to identify variants in USH genes;
[...] Read more.
Background: Usher syndrome (USH) is a rare genetic disorder that affects both hearing and vision. It presents in three clinical types—USH1, USH2, and USH3—with varying onset, severity, and disease progression. Existing diagnostics primarily rely on genetic profiling to identify variants in USH genes; however, accurate detection before symptom onset remains a challenge. MicroRNAs (miRNAs), which regulate gene expression, have been identified as potential biomarkers for disease. The aim of this study is to develop a data-driven system for the identification of USH using miRNA expression profiles. Methods: We collected microarray miRNA-expression data from 17 samples, representing four patient-derived USH cell lines and a non-USH control. Supervised feature selection was utilized to identify key miRNAs that differentiate USH cell lines from the non-USH control. Subsequently, a network model was constructed by measuring pairwise correlations based on these identified features. Results: The proposed system effectively distinguished between control and USH samples, demonstrating high accuracy. Additionally, the model could differentiate between the three USH types, reflecting its potential and sensitivity beyond the primary identification of affected subjects. Conclusions: This approach can be used to detect USH and differentiate between USH subtypes, suggesting its potential as a future base model for the identification of Usher syndrome.
Full article
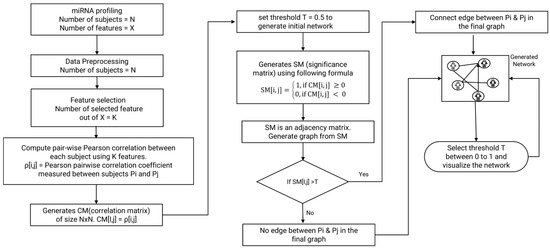
Figure 1
Open AccessReview
Systematic Review of Deep Learning Techniques in Skin Cancer Detection
by
Carolina Magalhaes, Joaquim Mendes and Ricardo Vardasca
BioMedInformatics 2024, 4(4), 2251-2270; https://doi.org/10.3390/biomedinformatics4040121 - 14 Nov 2024
Cited by 1
Abstract
►▼
Show Figures
Skin cancer is a serious health condition, as it can locally evolve into disfiguring states or metastasize to different tissues. Early detection of this disease is critical because it increases the effectiveness of treatment, which contributes to improved patient prognosis and reduced healthcare
[...] Read more.
Skin cancer is a serious health condition, as it can locally evolve into disfiguring states or metastasize to different tissues. Early detection of this disease is critical because it increases the effectiveness of treatment, which contributes to improved patient prognosis and reduced healthcare costs. Visual assessment and histopathological examination are the gold standards for diagnosing these types of lesions. Nevertheless, these processes are strongly dependent on dermatologists’ experience, with excision advised only when cancer is suspected by a physician. Multiple approaches have surfed over the last few years, particularly those based on deep learning (DL) strategies, with the goal of assisting medical professionals in the diagnosis process and ultimately diminishing diagnostic uncertainty. This systematic review focused on the analysis of relevant studies based on DL applications for skin cancer diagnosis. The qualitative assessment included 164 records relevant to the topic. The AlexNet, ResNet-50, VGG-16, and GoogLeNet architectures are considered the top choices for obtaining the best classification results, and multiclassification approaches are the current trend. Public databases are considered key elements in this area and should be maintained and improved to facilitate scientific research.
Full article
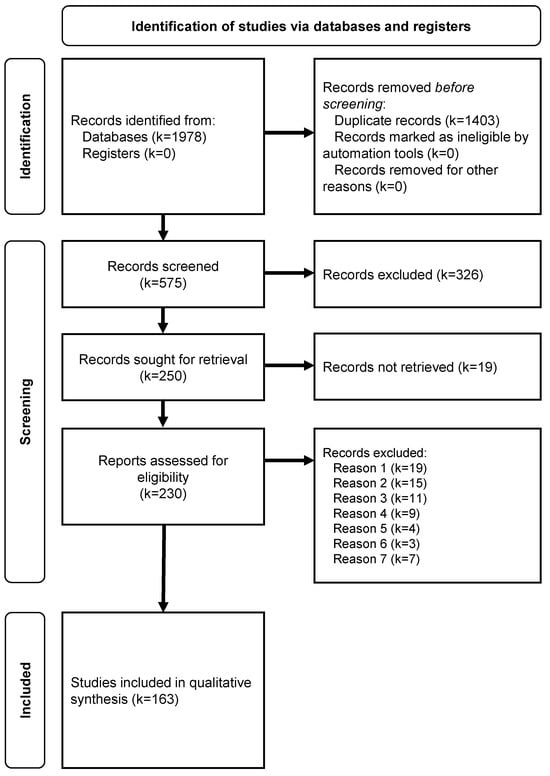
Figure 1
Open AccessArticle
Optimal Feature Selection and Classification for Parkinson’s Disease Using Deep Learning and Dynamic Bag of Features Optimization
by
Aarti, Swathi Gowroju, Mst Ismat Ara Begum and A. S. M. Sanwar Hosen
BioMedInformatics 2024, 4(4), 2223-2250; https://doi.org/10.3390/biomedinformatics4040120 - 12 Nov 2024
Abstract
►▼
Show Figures
Parkinson’s Disease (PD) is a neurological condition that worsens with time and is characterized bysymptoms such as cognitive impairment andbradykinesia, stiffness, and tremors. Parkinson’s is attributed to the interference of brain cells responsible for dopamine production, a substance regulating communication between brain cells.
[...] Read more.
Parkinson’s Disease (PD) is a neurological condition that worsens with time and is characterized bysymptoms such as cognitive impairment andbradykinesia, stiffness, and tremors. Parkinson’s is attributed to the interference of brain cells responsible for dopamine production, a substance regulating communication between brain cells. The brain cells involved in dopamine generation handle adaptation and control, and smooth movement. Convolutional Neural Networks are used to extract distinctive visual characteristics from numerous graphomotor sample representations generated by both PD and control participants. The proposed method presents an optimal feature selection technique based on Deep Learning (DL) and the Dynamic Bag of Features Optimization Technique (DBOFOT). Our method combines neural network-based feature extraction with a strong optimization technique to dynamically choose the most relevant characteristics from biological data. Advanced DL architectures are then used to classify the chosen features, guaranteeing excellent computational efficiency and accuracy. The framework’s adaptability to different datasets further highlights its versatility and potential for further medical applications. With a high accuracy of 0.93, the model accurately identifies 93% of the cases that are categorized as Parkinson’s. Additionally, it has a recall of 0.89, which means that 89% of real Parkinson’s patients are accurately identified. While the recall for Class 0 (Healthy) is 0.75, meaning that 75% of the real healthy cases are properly categorized, the precision decreases to 0.64 for this class, indicating a larger false positive rate.
Full article
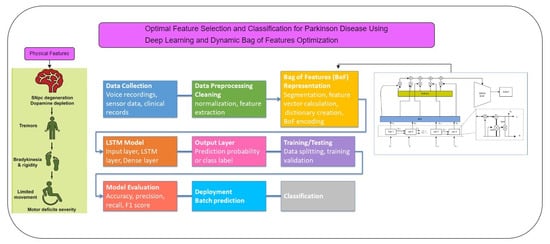
Graphical abstract
Open AccessArticle
Association Between Social Determinants of Health and Patient Portal Utilization in the United States
by
Elizabeth Ayangunna, Gulzar H. Shah, Hani Samawi, Kristie C. Waterfield and Ana M. Palacios
BioMedInformatics 2024, 4(4), 2213-2222; https://doi.org/10.3390/biomedinformatics4040119 - 12 Nov 2024
Abstract
(1) Background: Differences in health outcomes across populations are due to disparities in access to the social determinants of health (SDoH), such as educational level, household income, and internet access. With several positive outcomes reported with patient portal use, examining the associated social
[...] Read more.
(1) Background: Differences in health outcomes across populations are due to disparities in access to the social determinants of health (SDoH), such as educational level, household income, and internet access. With several positive outcomes reported with patient portal use, examining the associated social determinants of health is imperative. Objective: This study analyzed the association between social determinants of health—education, health insurance, household income, rurality, and internet access—and patient portal use among adults in the United States before and after the COVID-19 pandemic. (2) Methods: The research used a quantitative, retrospective study design and secondary data from the combined cycles 1 to 4 of the Health Information National Trends Survey 5 (N = 14,103) and 6 (N = 5958). Descriptive statistics and logistic regression were conducted to examine the association between the variables operationalizing SDoH and the use of patient portals. (3) Results: Forty-percent (40%) of respondents reported using a patient portal before the pandemic, and this increased to 61% in 2022. The multivariable logistic regression showed higher odds of patient portal utilization by women compared to men (AOR = 1.56; CI, 1.32–1.83), those with at least a college degree compared to less than high school education (AOR = 2.23; CI, 1.29–3.83), and annual family income of USD 75,000 and above compared to those <USD 20,000 (AOR = 1.59; CI, 1.18–2.15). Those with access to the internet and health insurance also had significantly higher odds of using their patient portals. However, those who identified as Hispanic and non-Hispanic Black and residing in a rural area rather than urban (AOR = 0.72; CI, 0.54–0.95) had significantly lower odds of using their patient portals even after the pandemic. (4) Conclusions: The social determinants of health included in this study showed significant influence on patient portal utilization, which has implications for policymakers and public health stakeholders tasked with promoting patient portal utilization and its benefits.
Full article
(This article belongs to the Special Issue Editor-in-Chief's Choices in Biomedical Informatics)
Open AccessArticle
Impact of Data Pre-Processing Techniques on XGBoost Model Performance for Predicting All-Cause Readmission and Mortality Among Patients with Heart Failure
by
Qisthi Alhazmi Hidayaturrohman and Eisuke Hanada
BioMedInformatics 2024, 4(4), 2201-2212; https://doi.org/10.3390/biomedinformatics4040118 - 1 Nov 2024
Abstract
►▼
Show Figures
Background: Heart failure poses a significant global health challenge, with high rates of readmission and mortality. Accurate models to predict these outcomes are essential for effective patient management. This study investigates the impact of data pre-processing techniques on XGBoost model performance in predicting
[...] Read more.
Background: Heart failure poses a significant global health challenge, with high rates of readmission and mortality. Accurate models to predict these outcomes are essential for effective patient management. This study investigates the impact of data pre-processing techniques on XGBoost model performance in predicting all-cause readmission and mortality among heart failure patients. Methods: A dataset of 168 features from 2008 heart failure patients was used. Pre-processing included handling missing values, categorical encoding, and standardization. Four imputation techniques were compared: Mean, Multivariate Imputation by Chained Equations (MICEs), k-nearest Neighbors (kNNs), and Random Forest (RF). XGBoost models were evaluated using accuracy, recall, F1-score, and Area Under the Curve (AUC). Robustness was assessed through 10-fold cross-validation. Results: The XGBoost model with kNN imputation, one-hot encoding, and standardization outperformed others, with an accuracy of 0.614, recall of 0.551, and F1-score of 0.476. The MICE-based model achieved the highest AUC (0.647) and mean AUC (0.65 ± 0.04) in cross-validation. All pre-processed models outperformed the default XGBoost model (AUC: 0.60). Conclusions: Data pre-processing, especially MICE with one-hot encoding and standardization, improves XGBoost performance in heart failure prediction. However, moderate AUC scores suggest further steps are needed to enhance predictive accuracy.
Full article
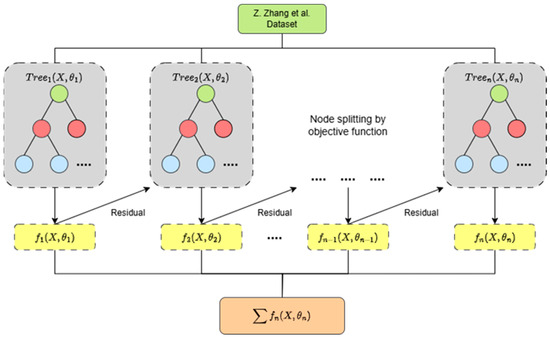
Figure 1
Open AccessArticle
Drosophila Eye Gene Regulatory Network Inference Using BioGRNsemble: An Ensemble-of-Ensembles Machine Learning Approach
by
Abdul Jawad Mohammed and Amal Khalifa
BioMedInformatics 2024, 4(4), 2186-2200; https://doi.org/10.3390/biomedinformatics4040117 - 29 Oct 2024
Abstract
Background: Gene regulatory networks (GRNs) are complex gene interactions essential for organismal development and stability, and they are crucial for understanding gene-disease links in drug development. Advances in bioinformatics, driven by genomic data and machine learning, have significantly expanded GRN research, enabling deeper
[...] Read more.
Background: Gene regulatory networks (GRNs) are complex gene interactions essential for organismal development and stability, and they are crucial for understanding gene-disease links in drug development. Advances in bioinformatics, driven by genomic data and machine learning, have significantly expanded GRN research, enabling deeper insights into these interactions. Methods: This study proposes and demonstrates the potential of BioGRNsemble, a modular and flexible approach for inferring gene regulatory networks from RNA-Seq data. Integrating the GENIE3 and GRNBoost2 algorithms, the BioGRNsemble methodology focuses on providing trimmed-down sub-regulatory networks consisting of transcription and target genes. Results: The methodology was successfully tested on a Drosophila melanogaster Eye gene expression dataset. Our validation analysis using the TFLink online database yielded 3703 verified predicted gene links, out of 534,843 predictions. Conclusion: Although the BioGRNsemble approach presents a promising method for inferring smaller, focused regulatory networks, it encounters challenges related to algorithm sensitivity, prediction bias, validation difficulties, and the potential exclusion of broader regulatory interactions. Improving accuracy and comprehensiveness will require addressing these issues through hyperparameter fine-tuning, the development of alternative scoring mechanisms, and the incorporation of additional validation methods.
Full article
(This article belongs to the Section Applied Biomedical Data Science)
►▼
Show Figures
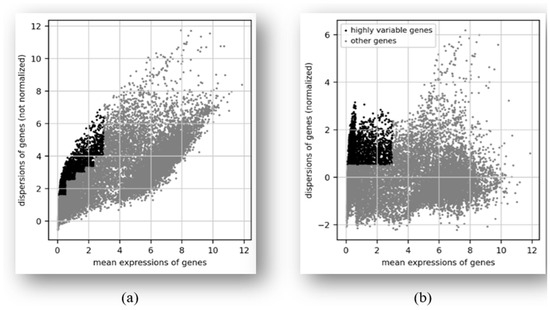
Figure 1
Highly Accessed Articles
Latest Books
E-Mail Alert
News
Topics
Topic in
BioMedInformatics, Cancers, Cells, Diagnostics, Immuno, IJMS
Inflammatory Tumor Immune Microenvironment
Topic Editors: William Cho, Anquan ShangDeadline: 15 March 2025
Topic in
Algorithms, BDCC, BioMedInformatics, Information, Mathematics
Machine Learning Empowered Drug Screen
Topic Editors: Teng Zhou, Jiaqi Wang, Youyi SongDeadline: 31 August 2025
Topic in
Applied Sciences, BioMedInformatics, BioTech, Genes, Computation
Computational Intelligence and Bioinformatics (CIB)
Topic Editors: Marco Mesiti, Giorgio Valentini, Elena Casiraghi, Tiffany J. CallahanDeadline: 30 September 2025
Topic in
AI, Algorithms, Applied Sciences, BioMedInformatics, Computers, Electronics
Theoretical Foundations and Applications of Deep Learning Techniques
Topic Editors: Juan Ernesto Solanes Galbis, Adolfo Muñoz GarcíaDeadline: 31 March 2026

Conferences
Special Issues
Special Issue in
BioMedInformatics
Advancements in Intelligent Natural Language Systems for Biomedical or Public Health Applications
Guest Editor: Susan McRoyDeadline: 30 June 2025
Special Issue in
BioMedInformatics
Editor-in-Chief's Choices in Biomedical Informatics
Guest Editor: Alexandre G. De BrevernDeadline: 25 July 2025
Special Issue in
BioMedInformatics
Editor's Choices Series for Clinical Informatics Section
Guest Editor: José MachadoDeadline: 31 July 2025
Special Issue in
BioMedInformatics
Integrating Health Informatics and Artificial Intelligence for Advanced Medicine
Guest Editor: Joaquim CarrerasDeadline: 31 August 2025