Journal Description
Physical Sciences Forum
Physical Sciences Forum
is an open access journal dedicated to publishing findings resulting from academic conferences, workshops and similar events in the area of physical sciences. Each conference proceeding can be individually indexed, is citable via a digital object identifier (DOI) and freely available under an open access license. The conference organizers and proceedings editors are responsible for managing the peer-review process and selecting papers for conference proceedings.
Latest Articles
Dual-Band Shared-Aperture Multimode OAM-Multiplexing Antenna Based on Reflective Metasurface
Phys. Sci. Forum 2024, 10(1), 6; https://doi.org/10.3390/psf2024010006 - 26 Dec 2024
Abstract
In this paper, a novel single-layer dual-band orbital angular momentum (OAM) multiplexed reflective metasurface array antenna is proposed, which can independently generate OAM beams with different modes in the C-band and Ku-band, and complete flexible beam control in each operating band, achieving the
[...] Read more.
In this paper, a novel single-layer dual-band orbital angular momentum (OAM) multiplexed reflective metasurface array antenna is proposed, which can independently generate OAM beams with different modes in the C-band and Ku-band, and complete flexible beam control in each operating band, achieving the generation of an OAM beam with mode l = −1 under oblique incidence at 7G with 94.4% mode purity, and having a wider usable operating bandwidth at 12G with a wide operating bandwidth, and an OAM beam with mode l = +2 is generated under oblique incidence, achieving 82.5% mode purity, which verifies the performance of the unit, makes preparations for the next research, and provides new possibilities for communication in more transmission bands and larger channel capacity.
Full article
(This article belongs to the Proceedings of The 1st International Online Conference on Photonics)
►
Show Figures
Open AccessProceeding Paper
Investigation the Optical Contrast Between Nanofiber Mats and Mammalian Cells Dyed with Fluorescent and Other Dyes
by
Nora Dassmann, Bennet Brockhagen and Andrea Ehrmann
Phys. Sci. Forum 2024, 10(1), 5; https://doi.org/10.3390/psf2024010005 - 26 Dec 2024
Abstract
Electrospinning can be used to prepare nanofiber mats from diverse polymers and polymer blends. A large area of research is the application of nanofibrous membranes for tissue engineering. Typically, cell adhesion and proliferation as well as the viability of mammalian cells are tested
[...] Read more.
Electrospinning can be used to prepare nanofiber mats from diverse polymers and polymer blends. A large area of research is the application of nanofibrous membranes for tissue engineering. Typically, cell adhesion and proliferation as well as the viability of mammalian cells are tested by seeding the cells on substrates, cultivating them for a defined time and finally dyeing them to enable differentiation between cells and substrates under a white light or fluorescence microscope. While this procedure works well for cells cultivated in well plates or petri dishes, other substrates may undesirably also be colored by the dye. Here we show investigations of the optical contrast between dyed CHO DP-12 (Chinese hamster ovary) cells and different electrospun nanofiber mats, dyed with haematoxylin-eosin (H&E), PromoFluor 488 premium, 4,6-diamidino-2-phenylindole (DAPI) or Hoechst 33342, and give the optimum dyeing parameters for maximum optical contrast between cells and nanofibrous substrates.
Full article
(This article belongs to the Proceedings of The 1st International Online Conference on Photonics)
►▼
Show Figures
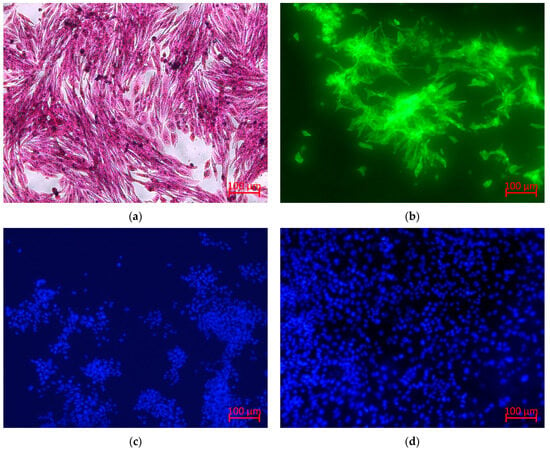
Figure 1
Open AccessProceeding Paper
Fast Method for the Measurement of Dispersion of Integrated Waveguides by Utilizing Michelson Interferometry Effects
by
Isaac Yorke, Lars Emil Gutt, Peter David Girouard and Michael Galili
Phys. Sci. Forum 2024, 10(1), 4; https://doi.org/10.3390/psf2024010004 - 20 Dec 2024
Abstract
In this paper we demonstrate a new approach to the measurement of dispersion of light reflected in integrated optical devices. The approach utilizes the fact that light reflected from the end facet of an integrated waveguide will interfere with light reflected from points
[...] Read more.
In this paper we demonstrate a new approach to the measurement of dispersion of light reflected in integrated optical devices. The approach utilizes the fact that light reflected from the end facet of an integrated waveguide will interfere with light reflected from points inside the device under test (DUT), effectively creating a Michelson interferometer. The distance between the measured fringes of this interferometric signal will depend directly on the group delay experienced in the device under test, allowing for fast and easy measurement of waveguide dispersion. This approach has been used to determine the dispersion of a fabricated linearly chirped Bragg gratings waveguide and the result agrees well with the designed value.
Full article
(This article belongs to the Proceedings of The 1st International Online Conference on Photonics)
►▼
Show Figures
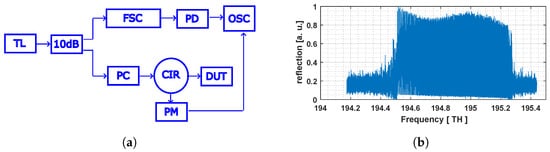
Figure 1
Open AccessProceeding Paper
Prototype of a Public Computer System with Fast Automatic Touchscreen Disinfection by Integrated UVC LEDs and Total Reflection
by
Sebastian Deuschl, Ben Sicks, Helge Moritz and Martin Hessling
Phys. Sci. Forum 2024, 10(1), 3; https://doi.org/10.3390/psf2024010003 - 17 Dec 2024
Abstract
Public touchscreens, such as those used in automated teller machines or ticket payment systems, which are accessed by different people in a short period of time, could transmit pathogens and thus spread infections. Therefore, the aim of this study was to develop and
[...] Read more.
Public touchscreens, such as those used in automated teller machines or ticket payment systems, which are accessed by different people in a short period of time, could transmit pathogens and thus spread infections. Therefore, the aim of this study was to develop and test a prototype of a touchscreen system for the public sector that disinfects itself quickly and automatically between two users without harming any humans. A quartz pane was installed in front of a commercial 19” monitor, into which 120 UVC LEDs emitted laterally. The quartz plate acted as a light guide and irradiated microorganisms on its surface, but—due to total reflection—not the user in front of the screen. A near-infrared commercial touch frame was installed to recognize touch. The antibacterial effect was tested through intentional staphylococcus contamination. The prototype, composed of a Raspberry Pi microcomputer with a display, a touchscreen, and a touch frame, was developed, and a simple game was programmed that briefly switched on the UVC LEDs between two users. The antimicrobial effect was so strong that 1% of the maximum UVC LED current was sufficient for a 99.9% staphylococcus reduction within 25 s. At 17.5% of the maximum current, no bacteria were observed after 5 s. The residual UVC irradiance at a distance of 100 mm in front of the screen was only 0.18 and 2.8 µW/cm2 for the two currents, respectively. This would allow users to stay in front of the system for 287 or 18 min, even if the LEDs were to emit UVC continuously and not be turned off after a few seconds as in the presented device. Therefore, fast, automatic touchscreen disinfection with UVC LEDs is already possible today, and with higher currents, disinfection durations below 1 s seems to be feasible, while the light guide approach virtually prevents the direct irradiation of the human user.
Full article
(This article belongs to the Proceedings of The 1st International Online Conference on Photonics)
►▼
Show Figures
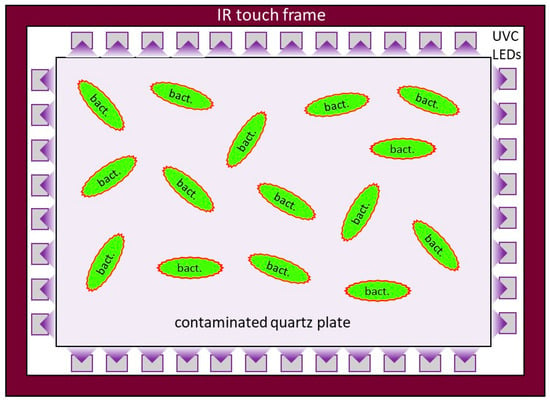
Figure 1
Open AccessProceeding Paper
Generation of Entangled Photon Pairs from High-Quality-Factor Silicon Microring Resonator at Near-Zero Anomalous Dispersion
by
Muneeb Farooq, Francisco Soares and Francisco Diaz
Phys. Sci. Forum 2024, 10(1), 2; https://doi.org/10.3390/psf2024010002 - 21 Nov 2024
Abstract
The intrinsic third-order nonlinearity in silicon has proven it to be quite useful in the field of quantum optics. Silicon is suitable for producing time-correlated photon pairs that are sources of heralded single-photon states for quantum integrated circuits. A quantum signal source in
[...] Read more.
The intrinsic third-order nonlinearity in silicon has proven it to be quite useful in the field of quantum optics. Silicon is suitable for producing time-correlated photon pairs that are sources of heralded single-photon states for quantum integrated circuits. A quantum signal source in the form of single photons is an inherent requirement for the principles of quantum key distribution technology for secure communications. Here, we present numerical simulations of a silicon ring with a
6
μ
m radius side-coupled with a bus waveguide as the source for the generation of single photons. The photon pairs are generated by exploring the process of degenerate spontaneous four-wave mixing (SFWM). The free spectral range (FSR) of the ring is quite large, simplifying the extraction of the signal/idler pairs. The phase-matching condition is considered by studying relevant parameters like the dispersion and nonlinearity. We optimize the ring for a high quality factor by varying the gap between the bus and the ring waveguide. This is the smallest ring studied for photon pair generation with a quality factor in the order of
10
5
. The width of the waveguides is chosen such that the phase-matching condition is satisfied, allowing for the propagation of fundamental modes only. The bus waveguide is pumped at one of the ring resonances with the minimum dispersion (1543.5 nm in our case) to satisfy the principle of energy conservation. The photon pair generation rate achieved is comparable to the state of the art. The photon pair sources exploiting nonlinear frequency conversion/generation processes is a promising alternative to atom-like single-photon emitters in the field of integrated photonics. Such miniaturized structures will benefit future on-chip architectures where multiple single-photon source devices are required on the same chip.
Full article
(This article belongs to the Proceedings of The 1st International Online Conference on Photonics)
►▼
Show Figures
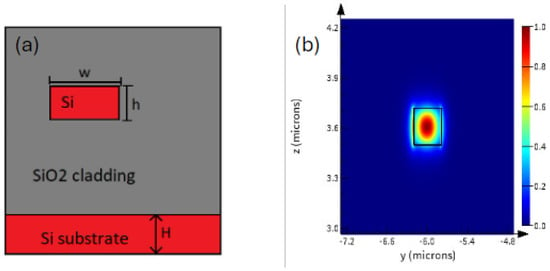
Figure 1
Open AccessProceeding Paper
Comparison of Different Far-UVC Sources with Regards to Intensity Stability, Estimated Antimicrobial Efficiency and Potential Human Hazard in Comparison to a Conventional UVC Lamp
by
Ben Sicks, Florian Maiss, Christian Lingenfelder, Cornelia Wiegand and Martin Hessling
Phys. Sci. Forum 2024, 10(1), 1; https://doi.org/10.3390/psf2024010001 - 19 Nov 2024
Abstract
The recently much noticed Far-UVC spectral range offers the possibility of inactivating pathogens without necessarily posing a major danger to humans. Unfortunately, there are various Far-UVC sources that differ significantly in their longer wavelength UVC emission and, subsequently, in their risk potential. Therefore,
[...] Read more.
The recently much noticed Far-UVC spectral range offers the possibility of inactivating pathogens without necessarily posing a major danger to humans. Unfortunately, there are various Far-UVC sources that differ significantly in their longer wavelength UVC emission and, subsequently, in their risk potential. Therefore, a simple assessment method for Far-UVC sources is presented here. In addition, the temporal intensity stability of Far-UVC sources was examined in order to reduce possible errors in irradiation measurements. For this purpose, four Far-UVC sources and a conventional Hg UVC lamp were each spectrally measured for about 100 h and mathematically evaluated for their antimicrobial effect and hazard potential using available standard data. The two filtered KrCl lamps were found to be most stable after a warm-up time of 30 min. With regard to the antimicrobial effect, the radiation efficiencies of all examined (Far-) UVC sources were more or less similar. However, the calculated differences in the potential human hazard to eyes and skin were more than one order of magnitude. The two filtered KrCl lamps were the safest, followed by an unfiltered KrCl lamp, a Far-UVC LED and, finally, the Hg lamp. When experimenting with these Far-UVC radiation sources, the irradiance should be checked more than once. If UVC radiation is to be or could be applied in the presence of humans, filtered KrCl lamps are a much better choice than any other available Far-UVC sources.
Full article
(This article belongs to the Proceedings of The 1st International Online Conference on Photonics)
►▼
Show Figures
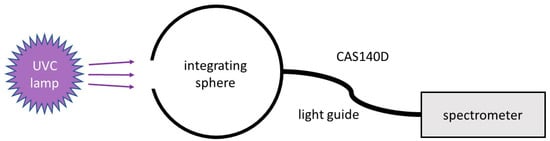
Figure 1
Open AccessProceeding Paper
Nested Sampling for Detection and Localization of Sound Sources Using a Spherical Microphone Array
by
Ning Xiang and Tomislav Jasa
Phys. Sci. Forum 2023, 9(1), 26; https://doi.org/10.3390/psf2023009026 - 20 May 2024
Abstract
Since its inception in 2004, nested sampling has been used in acoustics applications. This work applies nested sampling within a Bayesian framework to the detection and localization of sound sources using a spherical microphone array. Beyond an existing work, this source localization task
[...] Read more.
Since its inception in 2004, nested sampling has been used in acoustics applications. This work applies nested sampling within a Bayesian framework to the detection and localization of sound sources using a spherical microphone array. Beyond an existing work, this source localization task relies on spherical harmonics to establish parametric models that distinguish the background sound environment from the presence of sound sources. Upon a positive detection, the parametric models are also involved to estimate an unknown number of potentially multiple sound sources. For the purpose of source detection, a no-source scenario needs to be considered in addition to the presence of at least one sound source. Specifically, the spherical microphone array senses the sound environment. The acoustic data are analyzed via spherical Fourier transforms using a Bayesian model comparison of two different models accounting for the absence and presence of sound sources for the source detection. Upon a positive detection, potentially multiple source models are involved to analyze direction of arrivals (DoAs) using Bayesian model selection and parameter estimation for the sound source enumeration and localization. These are two levels (enumeration and localization) of inferential estimations necessary to correctly localize potentially multiple sound sources. This paper discusses an efficient implementation of the nested sampling algorithm applied to the sound source detection and localization within the Bayesian framework.
Full article
(This article belongs to the Proceedings of The 42nd International Workshop on Bayesian Inference and Maximum Entropy Methods in Science and Engineering)
►▼
Show Figures
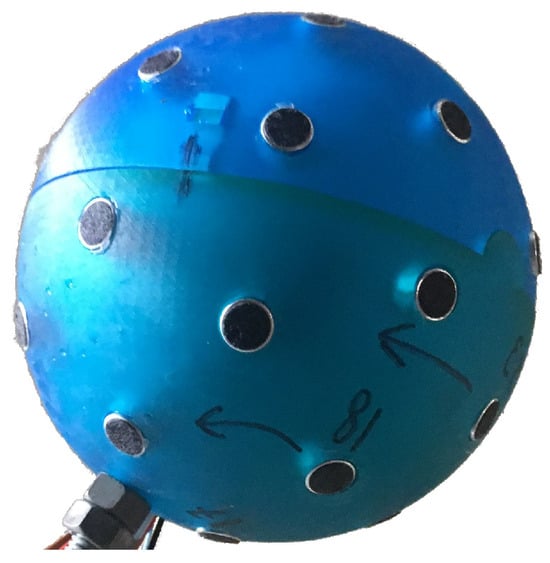
Figure 1
Open AccessProceeding Paper
Manifold-Based Geometric Exploration of Optimization Solutions
by
Guillaume Lebonvallet, Faicel Hnaien and Hichem Snoussi
Phys. Sci. Forum 2023, 9(1), 25; https://doi.org/10.3390/psf2023009025 - 16 May 2024
Abstract
This work introduces a new method for the exploration of solutions space in complex problems. This method consists of the build of a latent space which gives a new encoding of the solution space. We map the objective function on the latent space
[...] Read more.
This work introduces a new method for the exploration of solutions space in complex problems. This method consists of the build of a latent space which gives a new encoding of the solution space. We map the objective function on the latent space using a manifold, i.e., a mathematical object defined by an equations system. The latent space is built with some knowledge of the objective function to make the mapping of the manifold easier. In this work, we introduce a new encoding for the Travelling Salesman Problem (TSP) and we give a new method for finding the optimal round.
Full article
(This article belongs to the Proceedings of The 42nd International Workshop on Bayesian Inference and Maximum Entropy Methods in Science and Engineering)
►▼
Show Figures
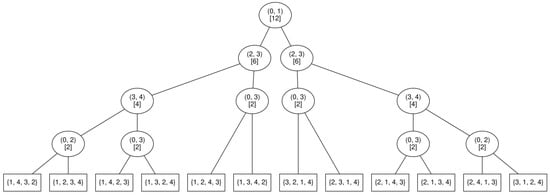
Figure 1
Open AccessProceeding Paper
NuMI Beam Monitoring Simulation and Data Analysis
by
Yiding Yu, Thomas Joseph Carroll, Sudeshna Ganguly, Karol Lang, Eduardo Ossorio, Pavel Snopok, Jennifer Thomas, Don Athula Wickremasinghe and Katsuya Yonehara
Phys. Sci. Forum 2023, 8(1), 73; https://doi.org/10.3390/psf2023008073 - 22 Apr 2024
Abstract
Following the decommissioning of the Main Injector Neutrino Oscillation Search (MINOS) experiment, muon and hadron monitors have emerged as vital diagnostic tools for the NuMI Off-axis Appearance (NOvA) experiment at Fermilab. These tools are crucial for overseeing the Neutrinos at the
[...] Read more.
Following the decommissioning of the Main Injector Neutrino Oscillation Search (MINOS) experiment, muon and hadron monitors have emerged as vital diagnostic tools for the NuMI Off-axis Appearance (NOvA) experiment at Fermilab. These tools are crucial for overseeing the Neutrinos at the Main Injector (NuMI) beam. This study endeavors to ensure the monitor signal quality and to correlate them with the Neutrino beam profile. Leveraging muon monitor simulations, we systematically explore the monitor responses to variations in proton-beam and lattice parameters. Through the amalgamation of individual pixel data from muon monitors, pattern-recognition algorithms, simulations, and measured data, we devise machine-learning-based models to predict muon monitor responses and Neutrino flux.
Full article
(This article belongs to the Proceedings of The 23rd International Workshop on Neutrinos from Accelerators)
►▼
Show Figures
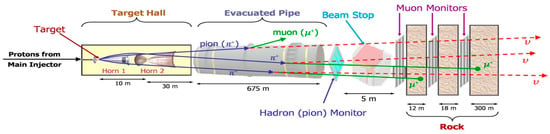
Figure 1
Open AccessProceeding Paper
Analysis of Ecological Networks: Linear Inverse Modeling and Information Theory Tools
by
Valérie Girardin, Théo Grente, Nathalie Niquil and Philippe Regnault
Phys. Sci. Forum 2023, 9(1), 24; https://doi.org/10.3390/psf2023009024 - 20 Feb 2024
Abstract
In marine ecology, the most studied interactions are trophic and are in networks called food webs. Trophic modeling is mainly based on weighted networks, where each weighted edge corresponds to a flow of organic matter between two trophic compartments, containing individuals of similar
[...] Read more.
In marine ecology, the most studied interactions are trophic and are in networks called food webs. Trophic modeling is mainly based on weighted networks, where each weighted edge corresponds to a flow of organic matter between two trophic compartments, containing individuals of similar feeding behaviors and metabolisms and with the same predators. To take into account the unknown flow values within food webs, a class of methods called Linear Inverse Modeling was developed. The total linear constraints, equations and inequations defines a multidimensional convex-bounded polyhedron, called a polytope, within which lie all realistic solutions to the problem. To describe this polytope, a possible method is to calculate a representative sample of solutions by using the Monte Carlo Markov Chain approach. In order to extract a unique solution from the simulated sample, several goal (cost) functions—also called Ecological Network Analysis indices—have been introduced in the literature as criteria of fitness to the ecosystems. These tools are all related to information theory. Here we introduce new functions that potentially provide a better fit of the estimated model to the ecosystem.
Full article
(This article belongs to the Proceedings of The 42nd International Workshop on Bayesian Inference and Maximum Entropy Methods in Science and Engineering)
Open AccessProceeding Paper
Development of a Clock Generation and Time Distribution System for Hyper-Kamiokande
by
Lucile Mellet, Mathieu Guigue, Boris Popov, Stefano Russo and Vincent Voisin
Phys. Sci. Forum 2023, 8(1), 72; https://doi.org/10.3390/psf2023008072 - 18 Jan 2024
Abstract
The construction of the next-generation water Cherenkov detector Hyper-Kamiokande (HK) has started. It will have about a ten times larger fiducial volume compared to the existing Super-Kamiokande detector, as well as increased detection performances. The data collection process is planned from 2027 onwards.
[...] Read more.
The construction of the next-generation water Cherenkov detector Hyper-Kamiokande (HK) has started. It will have about a ten times larger fiducial volume compared to the existing Super-Kamiokande detector, as well as increased detection performances. The data collection process is planned from 2027 onwards. Time stability is crucial, as detecting physics events relies on reconstructing Cherenkov rings based on the coincidence between the photomultipliers. The above requires a distributed clock jitter at each endpoint that is smaller than 100 ps. In addition, since this detector will be mainly used to detect neutrinos produced by the J-PARC accelerator in Tokai, each event needs to be timed-tagged with a precision better than 100 ns, with respect to UTC, in order to be associated with a proton spill from J-PARC or the events observed in other detectors for multi-messenger astronomy. The HK collaboration is in an R&D phase and several groups are working in parallel for the electronics system. This proceeding will present the studies performed at LPNHE (Paris) related to a novel design for the time synchronization system in Kamioka with respect to the previous KamiokaNDE series of experiments. We will discuss the clock generation, including the connection scheme between the GNSS receiver (Septentrio) and the atomic clock (free-running Rubidium), the precise calibration of the atomic clock and algorithms to account for errors on satellites orbits, the redundancy of the system, and a two-stage distribution system that sends the clock and various timing-sensitive information to each front-end electronics module, using a custom protocol.
Full article
(This article belongs to the Proceedings of The 23rd International Workshop on Neutrinos from Accelerators)
►▼
Show Figures
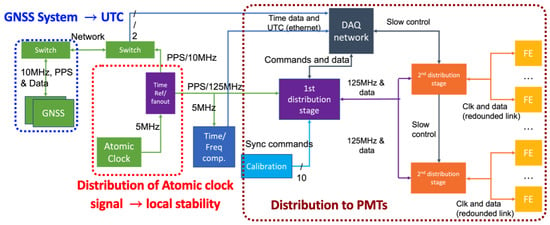
Figure 1
Open AccessProceeding Paper
Preconditioned Monte Carlo for Gradient-Free Bayesian Inference in the Physical Sciences
by
Minas Karamanis and Uroš Seljak
Phys. Sci. Forum 2023, 9(1), 23; https://doi.org/10.3390/psf2023009023 - 9 Jan 2024
Cited by 1
Abstract
We present preconditioned Monte Carlo (PMC), a novel Monte Carlo method for Bayesian inference in complex probability distributions. PMC incorporates a normalizing flow (NF) and an adaptive Sequential Monte Carlo (SMC) scheme, along with a novel past resampling scheme to boost the number
[...] Read more.
We present preconditioned Monte Carlo (PMC), a novel Monte Carlo method for Bayesian inference in complex probability distributions. PMC incorporates a normalizing flow (NF) and an adaptive Sequential Monte Carlo (SMC) scheme, along with a novel past resampling scheme to boost the number of propagated particles without extra computational costs. Additionally, we utilize preconditioned Crank–Nicolson updates, enabling PMC to scale to higher dimensions without the gradient of target distribution. The efficacy of PMC in producing samples, estimating model evidence, and executing robust inference is showcased through two challenging case studies, highlighting its superior performance compared to conventional methods.
Full article
(This article belongs to the Proceedings of The 42nd International Workshop on Bayesian Inference and Maximum Entropy Methods in Science and Engineering)
►▼
Show Figures
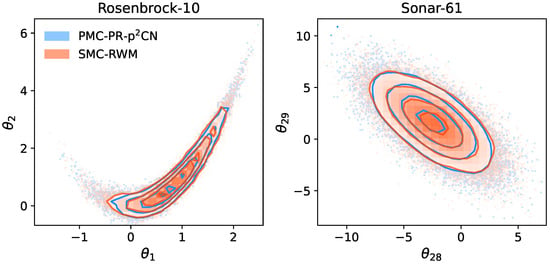
Figure 1
Open AccessProceeding Paper
Nested Sampling—The Idea
by
John Skilling
Phys. Sci. Forum 2023, 9(1), 22; https://doi.org/10.3390/psf2023009022 - 8 Jan 2024
Abstract
We seek to add up over unit volume in arbitrary dimension. Nested sampling locates the bulk of Q by geometrical compression, using a Monte Carlo ensemble constrained within a progressively more restrictive lower limit
[...] Read more.
We seek to add up over unit volume in arbitrary dimension. Nested sampling locates the bulk of Q by geometrical compression, using a Monte Carlo ensemble constrained within a progressively more restrictive lower limit . This domain is divided into a core and a shell , with the core kept adequately populated.
Full article
(This article belongs to the Proceedings of The 42nd International Workshop on Bayesian Inference and Maximum Entropy Methods in Science and Engineering)
►▼
Show Figures
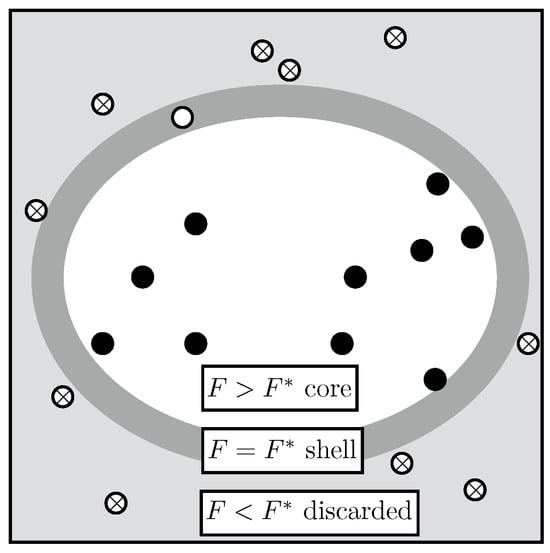
Figure 1
Open AccessProceeding Paper
Flow Annealed Kalman Inversion for Gradient-Free Inference in Bayesian Inverse Problems
by
Richard D. P. Grumitt, Minas Karamanis and Uroš Seljak
Phys. Sci. Forum 2023, 9(1), 21; https://doi.org/10.3390/psf2023009021 - 4 Jan 2024
Cited by 1
Abstract
For many scientific inverse problems, we are required to evaluate an expensive forward model. Moreover, the model is often given in such a form that it is unrealistic to access its gradients. In such a scenario, standard Markov Chain Monte Carlo algorithms quickly
[...] Read more.
For many scientific inverse problems, we are required to evaluate an expensive forward model. Moreover, the model is often given in such a form that it is unrealistic to access its gradients. In such a scenario, standard Markov Chain Monte Carlo algorithms quickly become impractical, requiring a large number of serial model evaluations to converge on the target distribution. In this paper, we introduce Flow Annealed Kalman Inversion (FAKI). This is a generalization of Ensemble Kalman Inversion (EKI) where we embed the Kalman filter updates in a temperature annealing scheme and use normalizing flows (NFs) to map the intermediate measures corresponding to each temperature level to the standard Gaussian. Thus, we relax the Gaussian ansatz for the intermediate measures used in standard EKI, allowing us to achieve higher-fidelity approximations to non-Gaussian targets. We demonstrate the performance of FAKI on two numerical benchmarks, showing dramatic improvements over standard EKI in terms of accuracy whilst accelerating its already rapid convergence properties (typically in steps).
Full article
(This article belongs to the Proceedings of The 42nd International Workshop on Bayesian Inference and Maximum Entropy Methods in Science and Engineering)
►▼
Show Figures
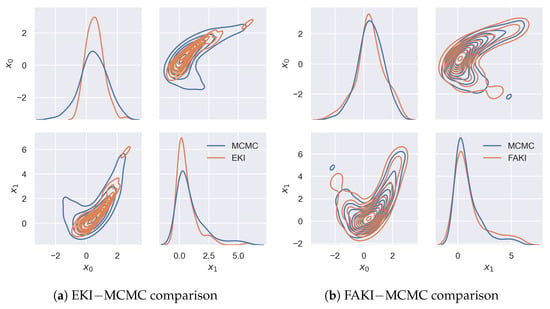
Figure 1
Open AccessProceeding Paper
Knowledge-Based Image Analysis: Bayesian Evidences Enable the Comparison of Different Image Segmentation Pipelines
by
Mats Leif Moskopp, Andreas Deussen and Peter Dieterich
Phys. Sci. Forum 2023, 9(1), 20; https://doi.org/10.3390/psf2023009020 - 4 Jan 2024
Abstract
The analysis and evaluation of microscopic image data is essential in life sciences. Increasing temporal and spatial digital image resolution and the size of data sets promotes the necessity of automated image analysis. Previously, our group proposed a Bayesian formalism that allows for
[...] Read more.
The analysis and evaluation of microscopic image data is essential in life sciences. Increasing temporal and spatial digital image resolution and the size of data sets promotes the necessity of automated image analysis. Previously, our group proposed a Bayesian formalism that allows for converting the experimenter’s knowledge, in the form of a manually segmented image, into machine-readable probability distributions of the parameters of an image segmentation pipeline. This approach preserved the level of detail provided by expert knowledge and interobserver variability and has proven robust to a variety of recording qualities and imaging artifacts. In the present work, Bayesian evidences were used to compare different image processing pipelines. As an illustrative example, a microscopic phase contrast image of a wound healing assay and its manual segmentation by the experimenter (ground truth) are used. Six different variations of image segmentation pipelines are introduced. The aim was to find the image segmentation pipeline that is best to automatically segment the input image given the expert knowledge with respect to the principle of Occam’s razor to avoid unnecessary complexity and computation. While none of the introduced image segmentation pipelines fail completely, it is illustrated that assessing the quality of the image segmentation with the naked eye is not feasible. Bayesian evidence (and the intrinsically estimated uncertainty of the image segmentation) is used to choose the best image processing pipeline for the given image. This work illustrates a proof of principle and is extendable to a diverse range of image segmentation problems.
Full article
(This article belongs to the Proceedings of The 42nd International Workshop on Bayesian Inference and Maximum Entropy Methods in Science and Engineering)
►▼
Show Figures
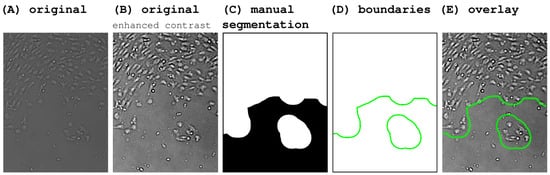
Figure 1
Open AccessProceeding Paper
Inferring Evidence from Nested Sampling Data via Information Field Theory
by
Margret Westerkamp, Jakob Roth, Philipp Frank, Will Handley and Torsten Enßlin
Phys. Sci. Forum 2023, 9(1), 19; https://doi.org/10.3390/psf2023009019 - 13 Dec 2023
Cited by 1
Abstract
Nested sampling provides an estimate of the evidence of a Bayesian inference problem via probing the likelihood as a function of the enclosed prior volume. However, the lack of precise values of the enclosed prior mass of the samples introduces probing noise, which
[...] Read more.
Nested sampling provides an estimate of the evidence of a Bayesian inference problem via probing the likelihood as a function of the enclosed prior volume. However, the lack of precise values of the enclosed prior mass of the samples introduces probing noise, which can hamper high-accuracy determinations of the evidence values as estimated from the likelihood-prior-volume function. We introduce an approach based on information field theory, a framework for non-parametric function reconstruction from data, that infers the likelihood-prior-volume function by exploiting its smoothness and thereby aims to improve the evidence calculation. Our method provides posterior samples of the likelihood-prior-volume function that translate into a quantification of the remaining sampling noise for the evidence estimate, or for any other quantity derived from the likelihood-prior-volume function.
Full article
(This article belongs to the Proceedings of The 42nd International Workshop on Bayesian Inference and Maximum Entropy Methods in Science and Engineering)
►▼
Show Figures
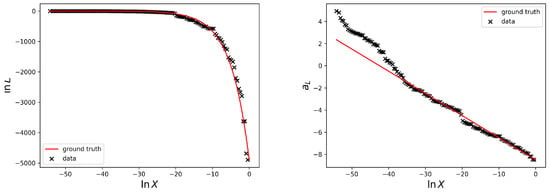
Figure 1
Open AccessProceeding Paper
A BRAIN Study to Tackle Image Analysis with Artificial Intelligence in the ALMA 2030 Era
by
Fabrizia Guglielmetti, Michele Delli Veneri, Ivano Baronchelli, Carmen Blanco, Andrea Dosi, Torsten Enßlin, Vishal Johnson, Giuseppe Longo, Jakob Roth, Felix Stoehr, Łukasz Tychoniec and Eric Villard
Phys. Sci. Forum 2023, 9(1), 18; https://doi.org/10.3390/psf2023009018 - 13 Dec 2023
Abstract
An ESO internal ALMA development study, BRAIN, is addressing the ill-posed inverse problem of synthesis image analysis, employing astrostatistics and astroinformatics. These emerging fields of research offer interdisciplinary approaches at the intersection of observational astronomy, statistics, algorithm development, and data science. In this
[...] Read more.
An ESO internal ALMA development study, BRAIN, is addressing the ill-posed inverse problem of synthesis image analysis, employing astrostatistics and astroinformatics. These emerging fields of research offer interdisciplinary approaches at the intersection of observational astronomy, statistics, algorithm development, and data science. In this study, we provide evidence of the benefits of employing these approaches to ALMA imaging for operational and scientific purposes. We show the potential of two techniques, RESOLVE and DeepFocus, applied to ALMA-calibrated science data. Significant advantages are provided with the prospect to improve the quality and completeness of the data products stored in the science archive and the overall processing time for operations. Both approaches evidence the logical pathway to address the incoming revolution in data rates dictated by the planned electronic upgrades. Moreover, we bring to the community additional products through a new package, ALMASim, to promote advancements in these fields, providing a refined ALMA simulator usable by a large community for training and testing new algorithms.
Full article
(This article belongs to the Proceedings of The 42nd International Workshop on Bayesian Inference and Maximum Entropy Methods in Science and Engineering)
►▼
Show Figures
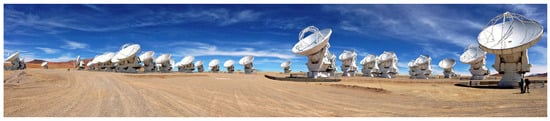
Figure 1
Open AccessProceeding Paper
Snowballing Nested Sampling
by
Johannes Buchner
Phys. Sci. Forum 2023, 9(1), 17; https://doi.org/10.3390/psf2023009017 - 6 Dec 2023
Abstract
A new way to run nested sampling, combined with realistic MCMC proposals to generate new live points, is presented. Nested sampling is run with a fixed number of MCMC steps. Subsequently, snowballing nested sampling extends the run to more and more live points.
[...] Read more.
A new way to run nested sampling, combined with realistic MCMC proposals to generate new live points, is presented. Nested sampling is run with a fixed number of MCMC steps. Subsequently, snowballing nested sampling extends the run to more and more live points. This stabilizes the MCMC proposal of later MCMC proposals, and leads to pleasant properties, including that the number of live points and number of MCMC steps do not have to be calibrated, that the evidence and posterior approximation improve as more compute is added and can be diagnosed with convergence diagnostics from the MCMC community. Snowballing nested sampling converges to a “perfect” nested sampling run with an infinite number of MCMC steps.
Full article
(This article belongs to the Proceedings of The 42nd International Workshop on Bayesian Inference and Maximum Entropy Methods in Science and Engineering)
►▼
Show Figures
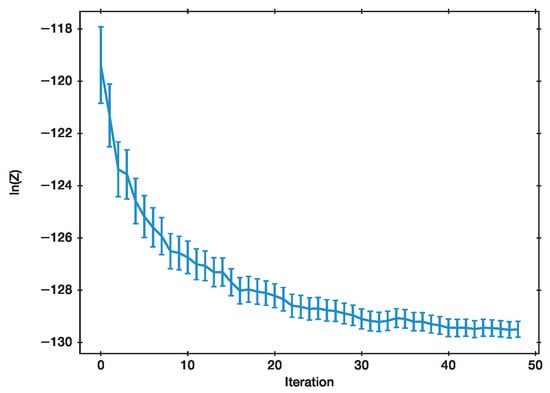
Figure 1
Open AccessProceeding Paper
Quantum Measurement and Objective Classical Reality
by
Vishal Johnson, Philipp Frank and Torsten Enßlin
Phys. Sci. Forum 2023, 9(1), 16; https://doi.org/10.3390/psf2023009016 - 6 Dec 2023
Abstract
We explore quantum measurement in the context of Everettian unitary quantum mechanics and construct an explicit unitary measurement procedure. We propose the existence of prior correlated states that enable this procedure to work and therefore argue that correlation is a resource that is
[...] Read more.
We explore quantum measurement in the context of Everettian unitary quantum mechanics and construct an explicit unitary measurement procedure. We propose the existence of prior correlated states that enable this procedure to work and therefore argue that correlation is a resource that is consumed when measurements take place. It is also argued that a network of such measurements establishes a stable objective classical reality.
Full article
(This article belongs to the Proceedings of The 42nd International Workshop on Bayesian Inference and Maximum Entropy Methods in Science and Engineering)
►▼
Show Figures
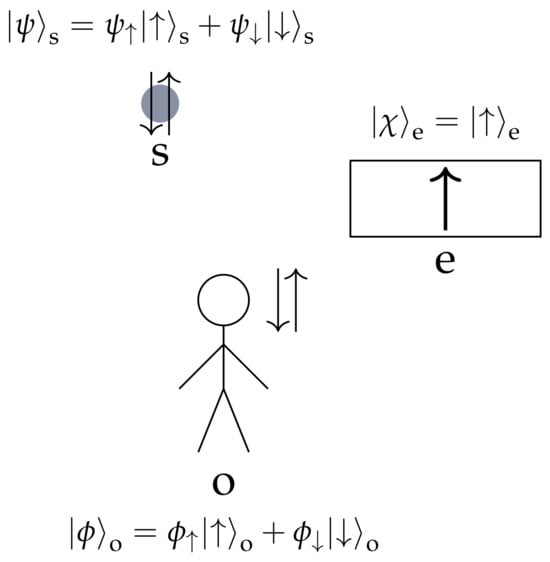
Figure 1
Open AccessProceeding Paper
Three-Dimensional Visualization of Astronomy Data Using Virtual Reality
by
Gilles Ferrand
Phys. Sci. Forum 2023, 8(1), 71; https://doi.org/10.3390/psf2023008071 - 5 Dec 2023
Abstract
Visualization is an essential part of research, both to explore one’s data and to communicate one’s findings with others. Many data products in astronomy come in the form of multi-dimensional cubes, and since our brains are tuned for recognition in a 3D world,
[...] Read more.
Visualization is an essential part of research, both to explore one’s data and to communicate one’s findings with others. Many data products in astronomy come in the form of multi-dimensional cubes, and since our brains are tuned for recognition in a 3D world, we ought to display and manipulate these in 3D space. This is possible with virtual reality (VR) devices. Drawing from our experiments developing immersive and interactive 3D experiences from actual science data at the Astrophysical Big Bang Laboratory (ABBL), this paper gives an overview of the opportunities and challenges that are awaiting astrophysicists in the burgeoning VR space. It covers both software and hardware matters, as well as practical aspects for successful delivery to the public.
Full article
(This article belongs to the Proceedings of The 23rd International Workshop on Neutrinos from Accelerators)
►▼
Show Figures
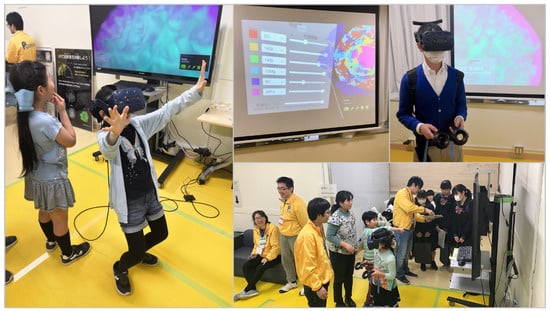
Figure 1
Highly Accessed Articles
Latest Books
E-Mail Alert
News
Topics
