Smart Sensors and Physics-Based Machine Learning for Structural Health Monitoring
A special issue of Sensors (ISSN 1424-8220). This special issue belongs to the section "Physical Sensors".
Deadline for manuscript submissions: closed (30 June 2022) | Viewed by 15241
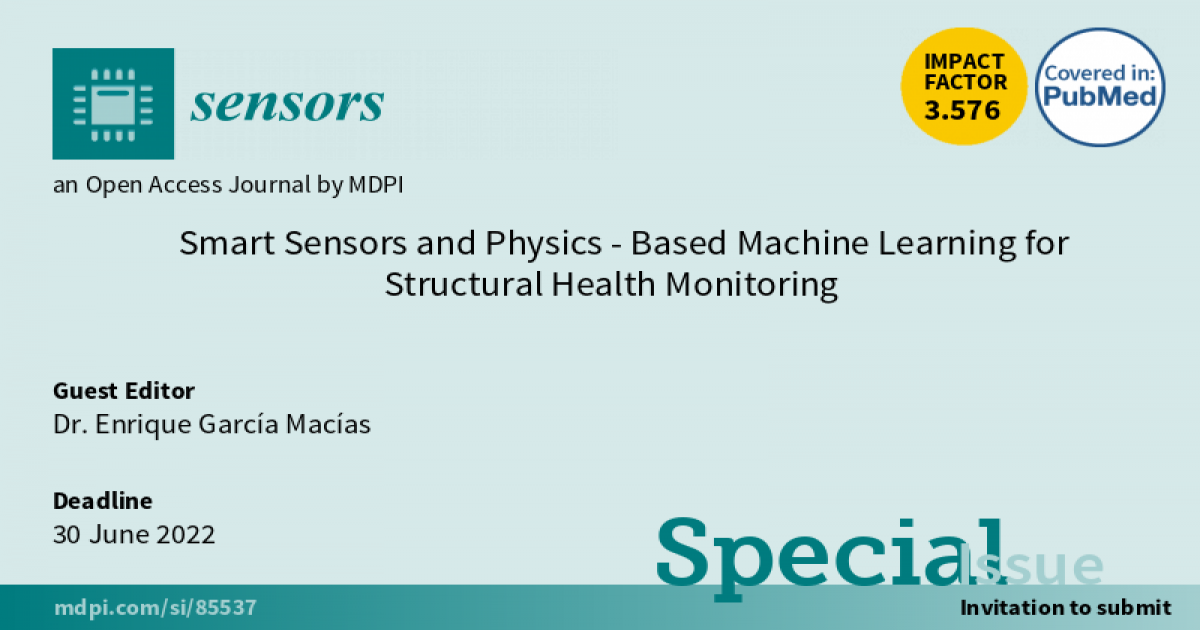
Special Issue Editor
Interests: structural health monitoring; operational modal analysis; multifunctional composite materials; system identification
Special Issues, Collections and Topics in MDPI journals
Special Issue Information
Dear Colleagues,
Recent advances in Smart Sensor Systems and Artificial Intelligence (AI) have opened vast possibilities for the development of disruptive innovations in the field of Structural Health Monitoring (SHM). In their broadest sense, smart sensors are designed to mitigate operating and efficiency limitations related to traditional monitoring solutions. These may range from sensors incorporating on-board microprocessing and state interrogation, sparse and dense sensor networks capable of detecting local and global pathologies, to novel composite materials with self-diagnostic properties. In addition, the increasingly frequent implementation of AI algorithms in the realm of SHM is enabling unprecedented possibilities to link monitoring signals to decision-making. Particularly promising are physics-based AI applications, enabling the injection of engineering knowledge and expertise into decision-making steps. In this light, the aim of this Special Issue is to generate discussions on the latest advances in research on smart sensing technologies and physics-based AI for SHM. Topics of interest include but are not limited to:
- Novel sensors and transducers;
- Intelligent signal processing;
- Smart sparse and dense sensor networks;
- Integrated systems;
- Multifunctional materials for sensing applications;
- Data fusion;
- Data mining;
- Supervised/unsupervised machine learning;
- Surrogate modeling for automated damage identification;
- Long-term big data processing and management;
- Internet of Things for structural health monitoring.
Dr. Enrique García Macías
Guest Editor
Manuscript Submission Information
Manuscripts should be submitted online at www.mdpi.com by registering and logging in to this website. Once you are registered, click here to go to the submission form. Manuscripts can be submitted until the deadline. All submissions that pass pre-check are peer-reviewed. Accepted papers will be published continuously in the journal (as soon as accepted) and will be listed together on the special issue website. Research articles, review articles as well as short communications are invited. For planned papers, a title and short abstract (about 100 words) can be sent to the Editorial Office for announcement on this website.
Submitted manuscripts should not have been published previously, nor be under consideration for publication elsewhere (except conference proceedings papers). All manuscripts are thoroughly refereed through a single-blind peer-review process. A guide for authors and other relevant information for submission of manuscripts is available on the Instructions for Authors page. Sensors is an international peer-reviewed open access semimonthly journal published by MDPI.
Please visit the Instructions for Authors page before submitting a manuscript. The Article Processing Charge (APC) for publication in this open access journal is 2600 CHF (Swiss Francs). Submitted papers should be well formatted and use good English. Authors may use MDPI's English editing service prior to publication or during author revisions.
Benefits of Publishing in a Special Issue
- Ease of navigation: Grouping papers by topic helps scholars navigate broad scope journals more efficiently.
- Greater discoverability: Special Issues support the reach and impact of scientific research. Articles in Special Issues are more discoverable and cited more frequently.
- Expansion of research network: Special Issues facilitate connections among authors, fostering scientific collaborations.
- External promotion: Articles in Special Issues are often promoted through the journal's social media, increasing their visibility.
- e-Book format: Special Issues with more than 10 articles can be published as dedicated e-books, ensuring wide and rapid dissemination.
Further information on MDPI's Special Issue polices can be found here.