Volume Ⅱ: Advances in Wind and Solar Farm Forecasting
A special issue of Energies (ISSN 1996-1073). This special issue belongs to the section "A3: Wind, Wave and Tidal Energy".
Deadline for manuscript submissions: closed (30 April 2024) | Viewed by 16300
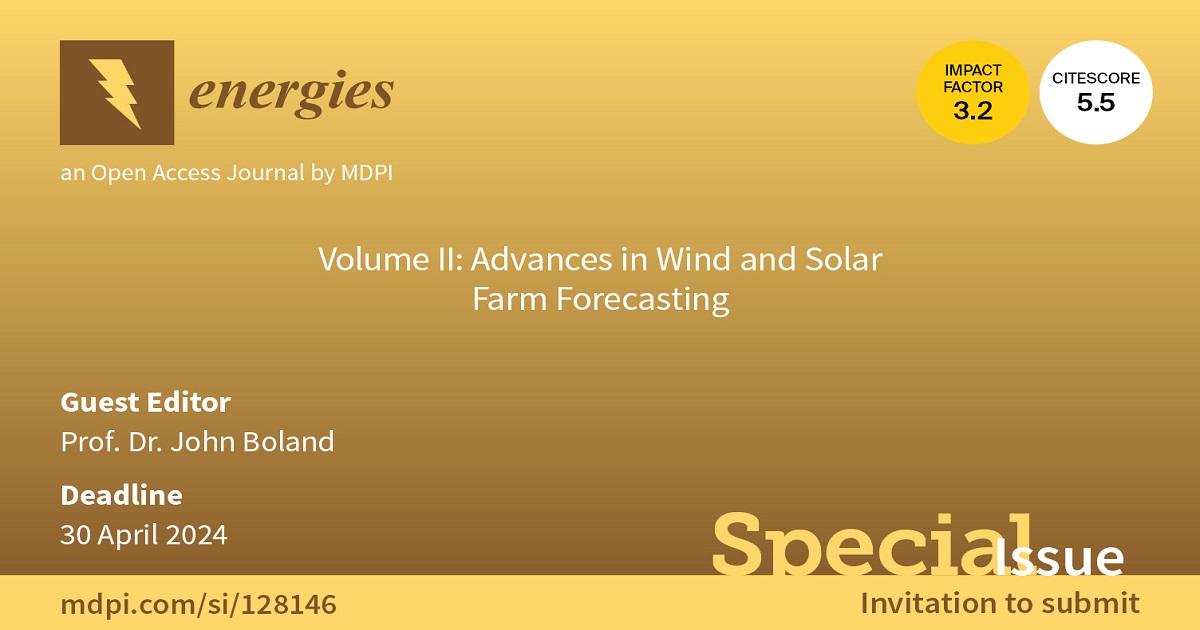
Special Issue Editor
Interests: time series analysis and forecasting for climate variables; renewable energy utilization; climate change and risk analysis; heat transfer and energy efficient buildings; water harvesting; ecological footprint; sustainable diet
Special Issues, Collections and Topics in MDPI journals
Special Issue Information
Dear Colleagues,
Intermittent electrical power output from grid-connected solar and wind farms increases the difficulty of managing and maintaining electricity grid stability. The difficulty arises from the uncertainty of the electrical power output from the farms, adversely affecting the control of dispatchable power to balance power supply and demand. Given the high rate of growth of these installations, and the majority of research in forecasting focussed on the resource, it is expedient to turn our attention more to the direct forecasting of output from both wind and solar farms. Additionally, it is extremely important to not only home in on point forecasting, but also to explore robust techniques for probabilistic forecasting. Allied to these topics is the issue of identifying the value of forecasts, both point and probabilistic.
Topics will include:
- Point forecasting methods for wind or solar farm output
- Probabilistic forecasting
- Value of forecasting
- Classical time series methods
- Physical forecasting methods
- Satellite image tools
- Machine learning methods
- Numerical weather prediction
- Blended forecasting tools
- Spatiotemporal forecasting
Prof. Dr. John Boland
Guest Editor
Manuscript Submission Information
Manuscripts should be submitted online at www.mdpi.com by registering and logging in to this website. Once you are registered, click here to go to the submission form. Manuscripts can be submitted until the deadline. All submissions that pass pre-check are peer-reviewed. Accepted papers will be published continuously in the journal (as soon as accepted) and will be listed together on the special issue website. Research articles, review articles as well as short communications are invited. For planned papers, a title and short abstract (about 100 words) can be sent to the Editorial Office for announcement on this website.
Submitted manuscripts should not have been published previously, nor be under consideration for publication elsewhere (except conference proceedings papers). All manuscripts are thoroughly refereed through a single-blind peer-review process. A guide for authors and other relevant information for submission of manuscripts is available on the Instructions for Authors page. Energies is an international peer-reviewed open access semimonthly journal published by MDPI.
Please visit the Instructions for Authors page before submitting a manuscript. The Article Processing Charge (APC) for publication in this open access journal is 2600 CHF (Swiss Francs). Submitted papers should be well formatted and use good English. Authors may use MDPI's English editing service prior to publication or during author revisions.
Keywords
- deterministic output forecasting
- probabilistic forecasting
- value of forecasting
- minimised curtailment
Benefits of Publishing in a Special Issue
- Ease of navigation: Grouping papers by topic helps scholars navigate broad scope journals more efficiently.
- Greater discoverability: Special Issues support the reach and impact of scientific research. Articles in Special Issues are more discoverable and cited more frequently.
- Expansion of research network: Special Issues facilitate connections among authors, fostering scientific collaborations.
- External promotion: Articles in Special Issues are often promoted through the journal's social media, increasing their visibility.
- e-Book format: Special Issues with more than 10 articles can be published as dedicated e-books, ensuring wide and rapid dissemination.
Further information on MDPI's Special Issue polices can be found here.
Related Special Issues
- Advances in Wind and Solar Farm Forecasting in Energies (8 articles)
- Advances in Wind and Solar Farm Forecasting—3rd Edition in Energies (3 articles)